The future of 'standard' medical practice is about to change with Artificial intelligence. Imagine you walk into a healthcare facility and, before you even chat with a doctor, you're greeted by a computer.
Thanks to advances in artificial intelligence (AI), we're so much closer to a world where misdiagnosis becomes a thing of the past, and we focus on nipping diseases in the bud, not just battling their symptoms.
Have you ever wondered how many blood pressure measurements have been recorded over the years? Or the massive amount of storage you'd need to stash a complete 3D image of an organ on your laptop?
With datasets piling up in clinics and getting neatly filed away in electronic medical records, we're seeing more and more artificial intelligence applications in healthcare.
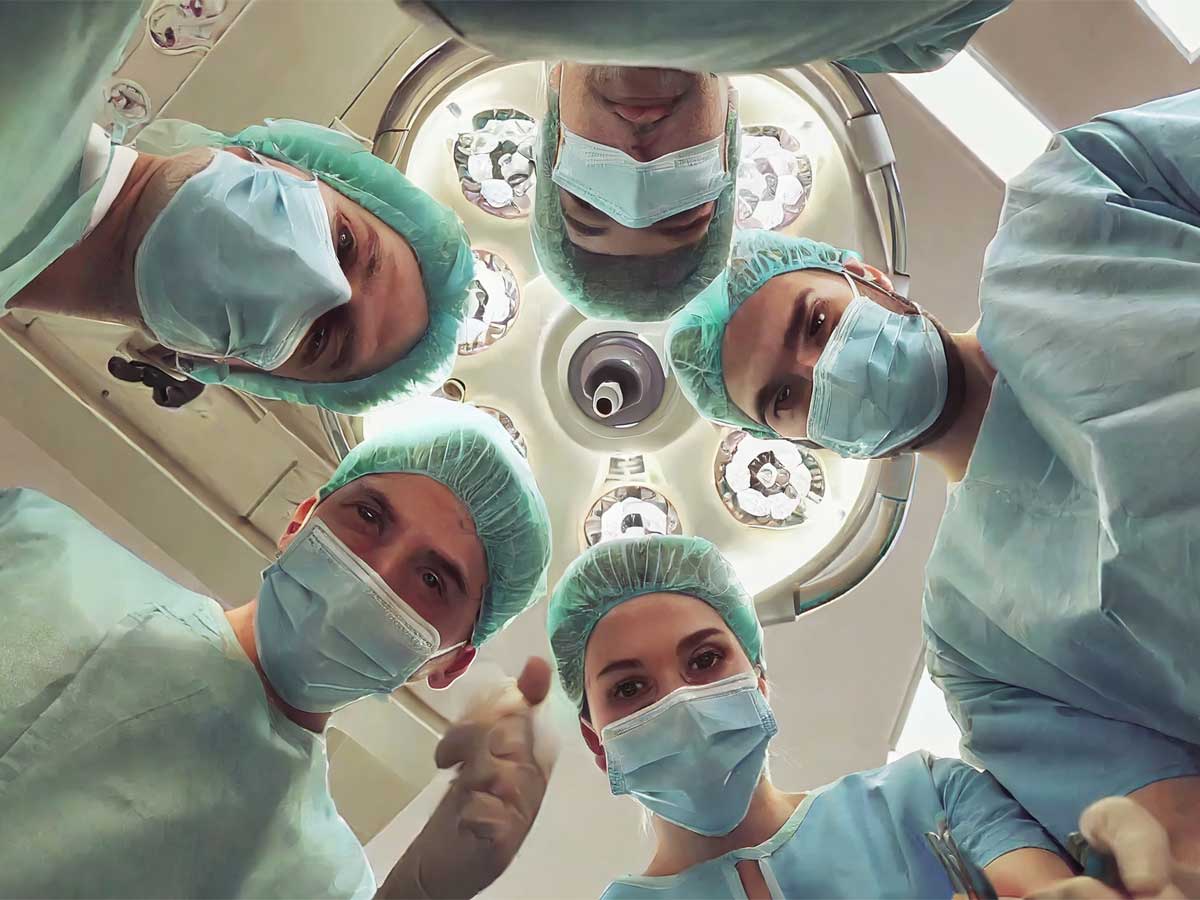
This is what high-performance, data-driven medicine is all about. Both doctors and researchers are leveraging this tech to revolutionize how they solve clinical problems.
But even though some algorithms can match or even outdo clinicians in various tasks, they still need to be fully woven into our everyday medical routines. Well, as powerful as these algorithms are in transforming medical care, there are quite a few regulatory concerns to iron out first. I will explain everything, but let's start from the beginning.
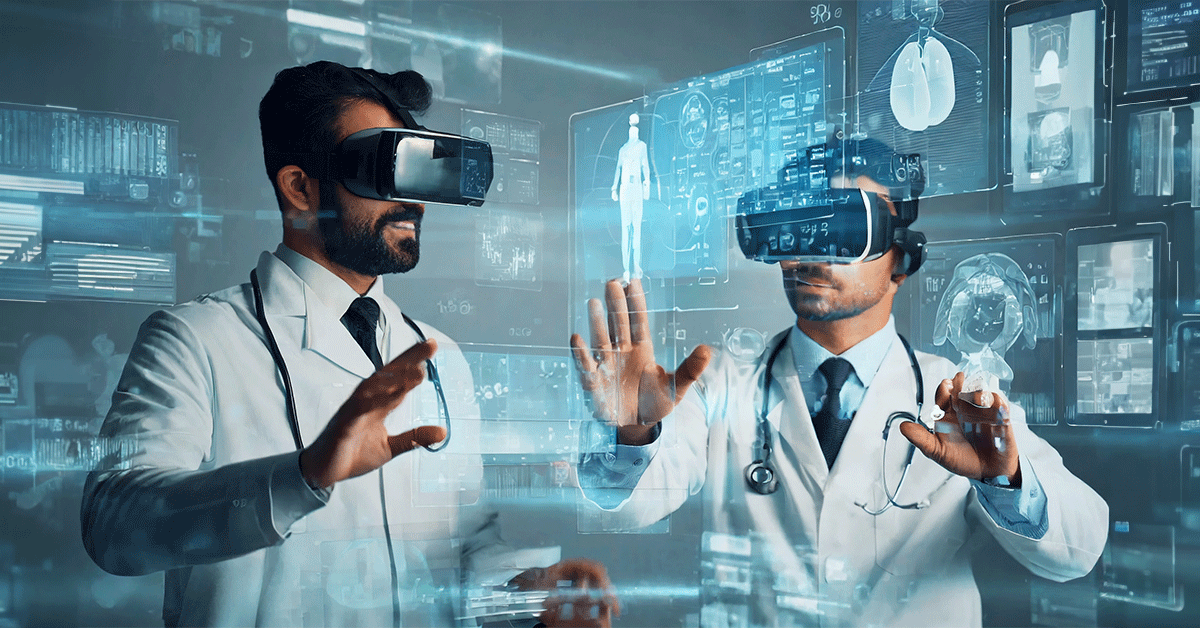
Algorithmic Applications of Artificial Intelligence in Medicine
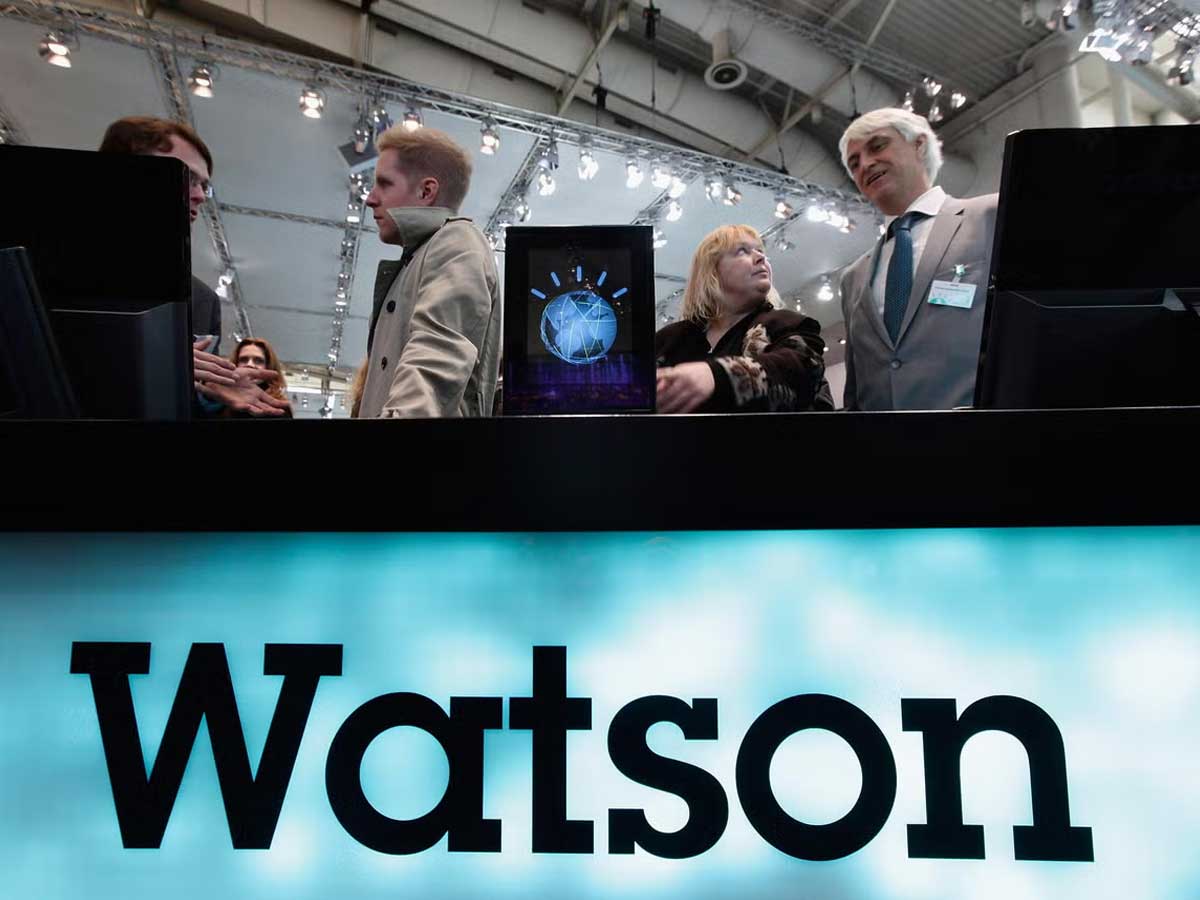
Just like medical students learn through schooling and exams, algorithms become smart by going through a similar learning process. It's fascinating how AI algorithms are being trained to do stuff that usually needs a human touch, like pattern recognition, speech recognition, image analysis, and even making decisions. Read our recent article about 'Convolutional Neural Network (CNN) & Deep Learning.'
End of the day, humans set the rules for these AI algorithms so they know exactly what to look for in images or how to tackle specific tasks.
What is Machine learning (ML) and Natural Language Processing (NLP)
Machine learning is basically a branch of artificial intelligence.
Artificial intelligence is a machine's ability to mimic how the human brain works and intelligence. Machine learning is a part of AI that uses algorithms to teach computers to learn and improve their processes.
Machine learning algorithms have the ability to enhance their performance over time as they are trained with large amounts of data. These algorithms generate machine learning models that contain the knowledge gained from running algorithms on training data.
The more data that is used to train the algorithm, the more accurate and refined the model will become.
Natural Language Processing (NLP)
In natural language processing, machine learning models empower virtual assistants such as Alexa, Google Assistant, and Siri to comprehend and respond to human language.
Now, these AI algorithms are capable of handling complex tasks, often doing them better than us humans in the areas they're trained in. To get them up to speed, computer systems feed them structured data neatly labeled and organized.
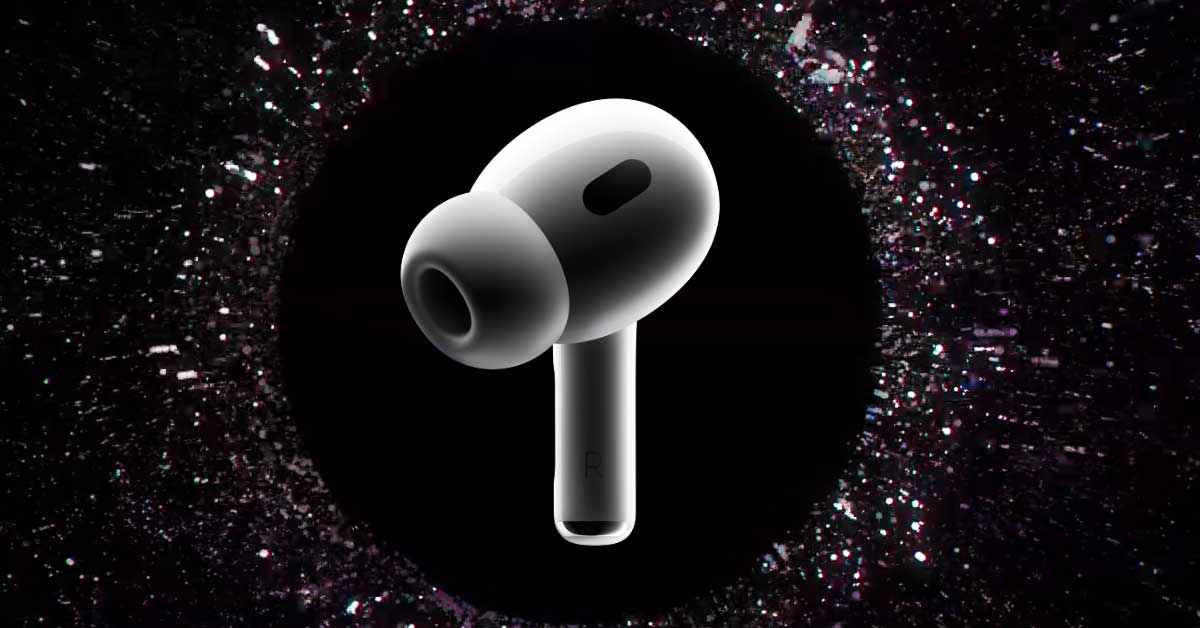
A whole variety of algorithms are out there learning from all sorts of data. Some work on numbers like heart rate and blood pressure, while others train from images, like MRI scans or biopsy tissue samples. They put things like probabilities (like the odds of having an arterial clot based on your heart rate) or categorizing stuff (like whether a tissue sample is cancerous or not).
Physician-Machine Collaboration in Medicine
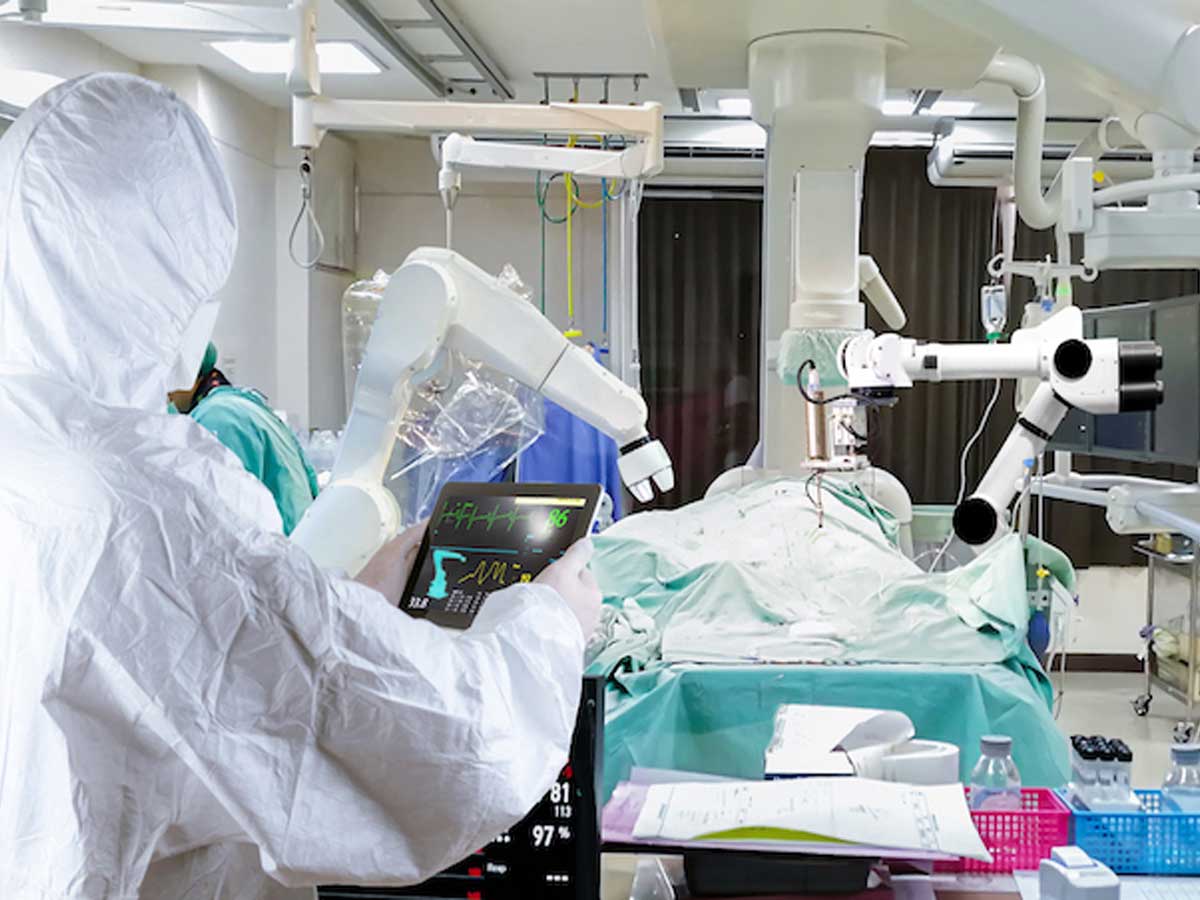
In healthcare, there's a buzz about how physician-machine collaboration might outdo what doctors or machines can do on their own. Especially in areas like radiology, pathology, and dermatology, the smarts of AI could rival or even beat the expertise of clinicians.
But don't think AI's about to take over doctors' jobs! The human touch - empathy, compassion, and savvy decision-making - remains key in patient care.
The American Medical Association is all for using tech to boost, not boot out, human smarts. And here's a neat thing: AI might just ease up physician burnout by taking over the yawn-inducing admin tasks so docs can focus more on looking after us. Plus, it could help fix the shortage of healthcare workers and make healthcare more accessible.
As AI keeps improving, physicians might shift towards big-picture thinking, connecting with patients, and working together across different fields.
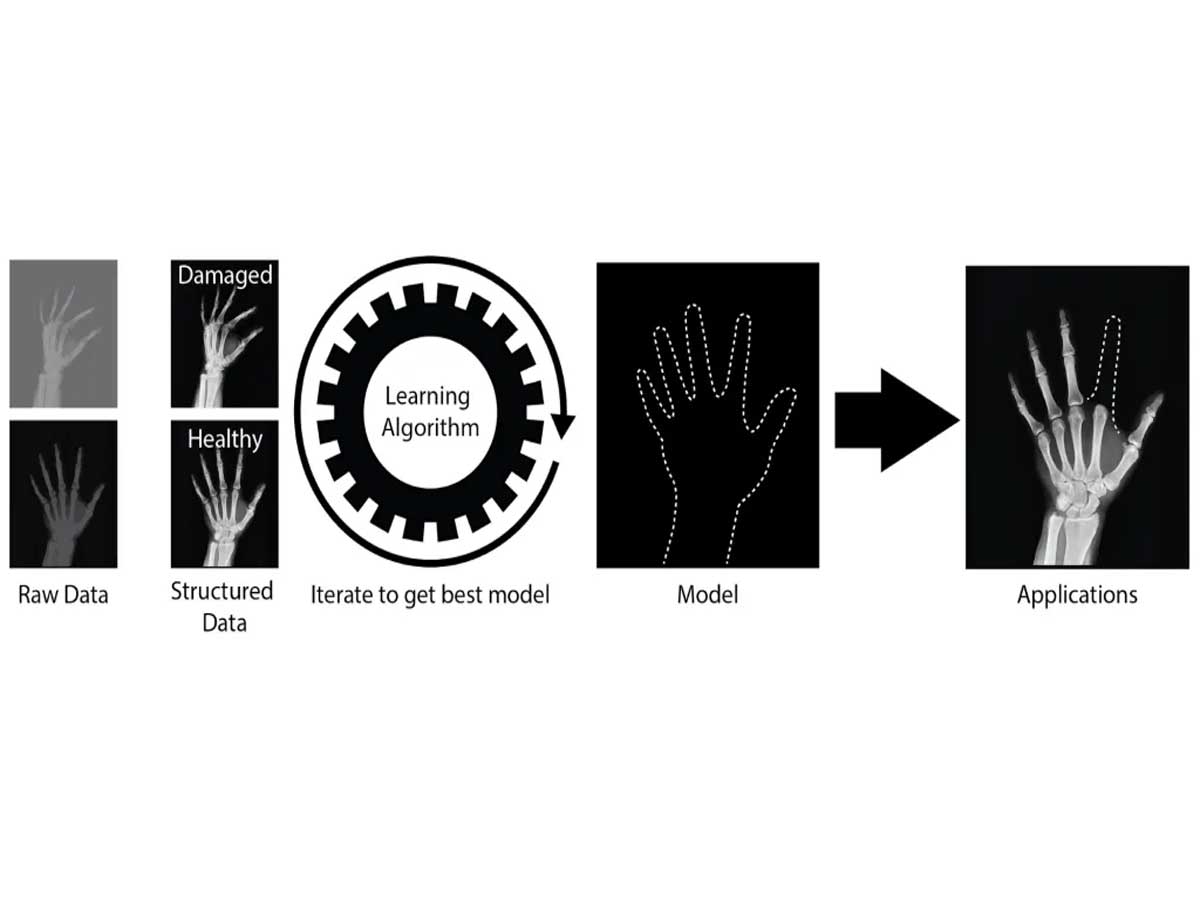
Physicians have a huge part to play in making sure all the decisions are ethical and that patients get top-notch care. They've got to be ready for new challenges and roles in this AI boom.
AI's got the potential to improve health care- better patient outcomes, amazing discoveries, and breakthroughs in stopping or treating diseases, thanks to its ability to crunch tons of data. But, fitting AI into everyday clinical stuff needs careful testing, training, and constant checks to ensure it's safe and doing its job right.
AI applications in medicine
AI in medicine takes disease detection and diagnosis to another level. AI keeps an eye on critical care patients' vital signs, ready to flag any sepsis risk factors to the doctors. And it's not just basic monitoring; these smart systems can sift through data from devices like heart monitors to spot even the tricky things to notice by human eyes.
There's this cool development from an IBM client – they've made a predictive AI model with a 75% hit rate in spotting severe sepsis in premature babies. And then there's personalized disease treatment.
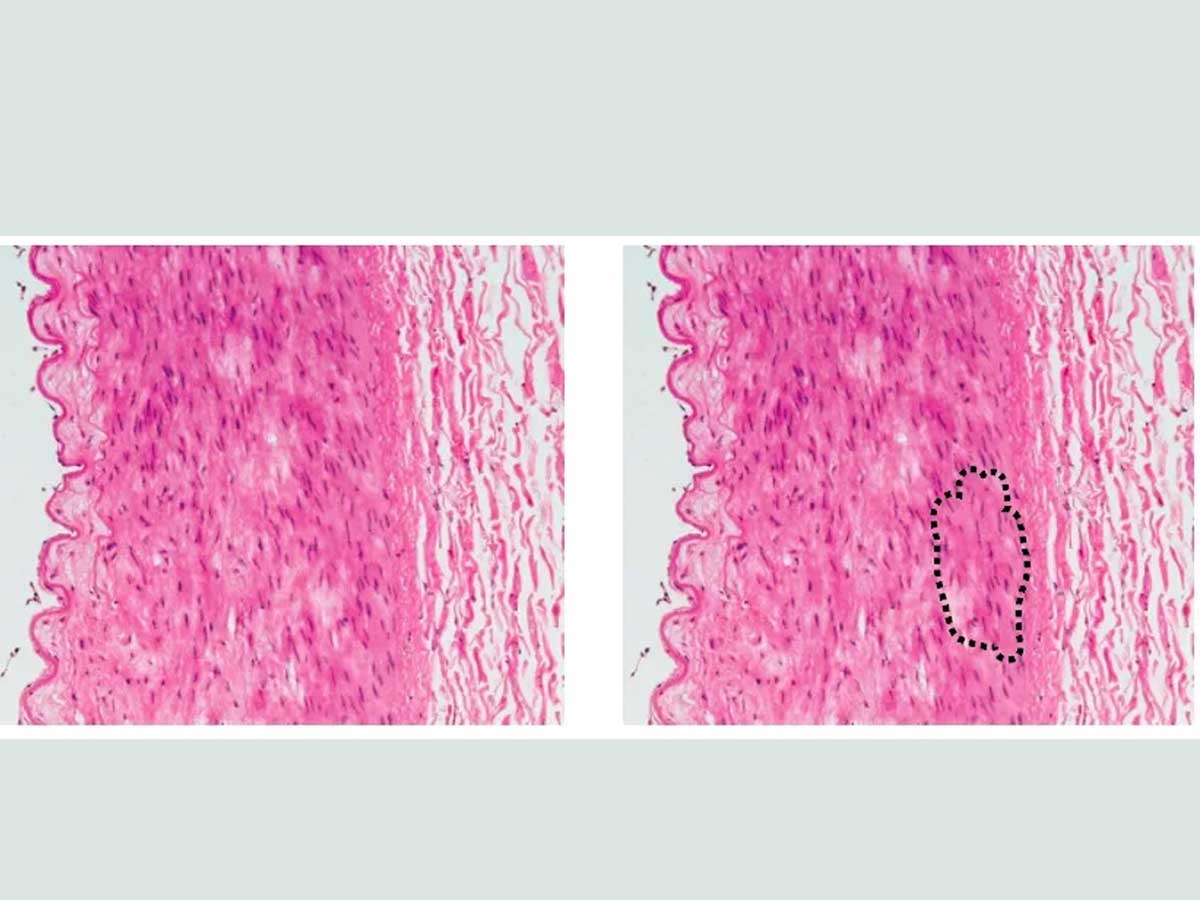
We talked about how the artificial intelligence assistant works right. An AI-powered virtual assistant who knows your medical history and preferences inside out could offer you proper health advice around the clock. There have been several incidents in which lives have been saved because of smart devices.
AI in medical imaging is with neck and neck with human radiologists in catching signs of breast cancer and other conditions. Plus, it's a huge help for clinicians drowning in a sea of medical images, picking out the ones that really matter.
When it comes to clinical trial efficiency, AI is very helpful. It's reducing the time spent on medical code searches by more than 70%.
What Ever Happened to IBM’s Watson?
IBM Watson was once considered the future of healthcare. However, it failed to live up to its promise. Some reasons for its failure include:
- Data: Watson's cancer diagnostics tool was trained with hypothetical cases instead of real patient data. The cancer data was also too complex and tainted by misdiagnoses and doctors' expressions.
- Target customers: Watson's target customers were overworked doctors who already had too much administrative work.
- Lack of data: There needed to be more data for the program to make good recommendations.
In 2022, IBM sold parts of its Watson Health division to Francisco Partners. The Watson Health division was then spun into Merative, a standalone company headquartered in Ann Arbor.
IBM has said it will continue developing and supporting Watson for Oncology and other products.
And let's not forget accelerated drug development. Here, AI is the brains behind creating better drug designs, uncovering promising new drug combos, and, at the same time, reducing the cost of medicine and health treatments.
Benefits of AI in medicine
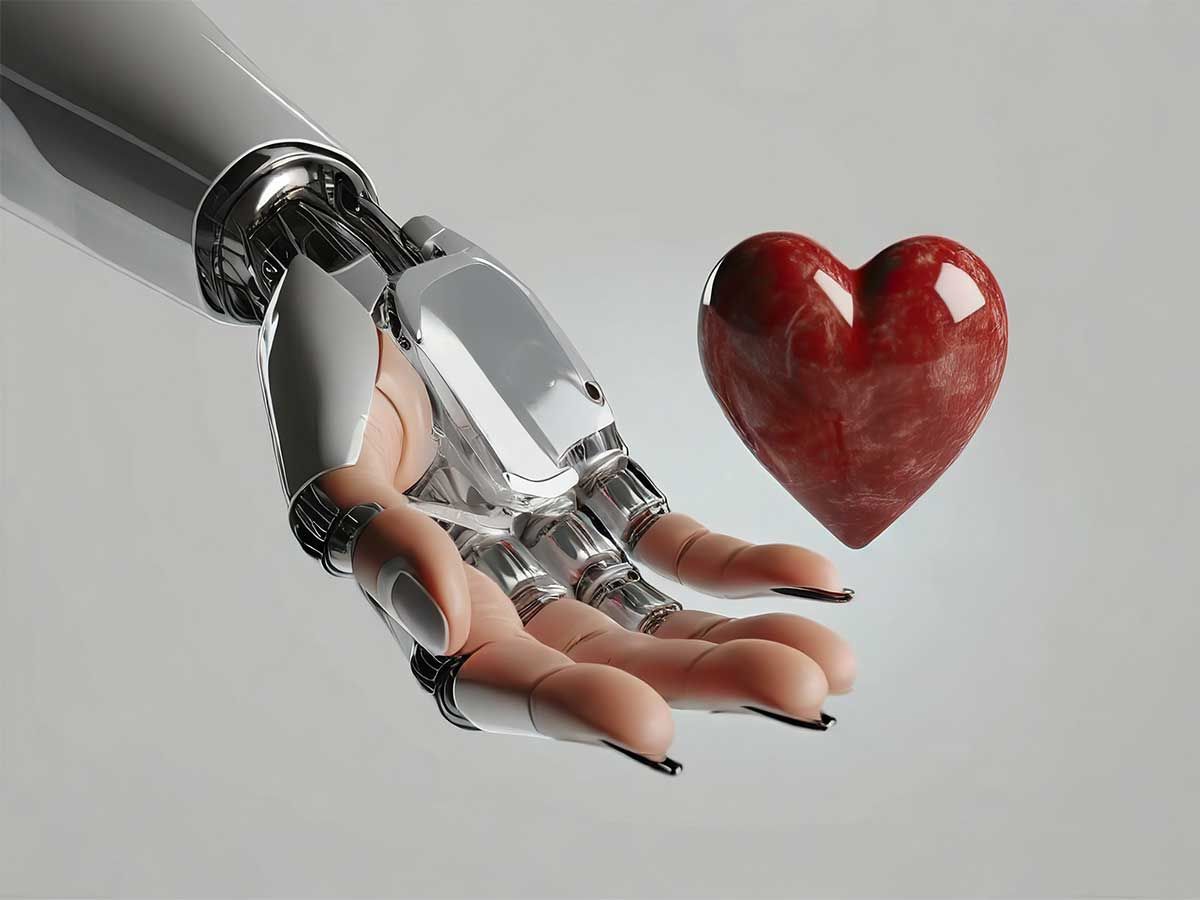
Informed patient care: integrating medical AI into clinician workflows is a game-changer. It arms healthcare professionals with a trained machine learning algorithm that significantly reduces research time.
This means doctors get to provide care based on solid, evidence-based insights about treatments and procedures, all while the patient is still there with them.
Error reduction: there's growing evidence that Artificial intelligence is a big win for patient safety. A recent systemic review of 53 peer-reviewed studies highlights the impact of AI on patient safety. AI-powered decision support tools are particularly good at improving error detection and drug management.
Reducing the costs of care: Intelligence systems show promising potential. From reducing medication errors to customized virtual health assistance, This can streamline many aspects of healthcare. It's also a tool in fraud prevention and supporting efficient administrative and clinical workflows, contributing to cost savings.
Increasing doctor-patient engagement: AI-powered systems offer around-the-clock support with chatbots that can answer basic health questions anytime. This tech is particularly handy when the provider's office is closed, helping triage questions and flagging information for further review.
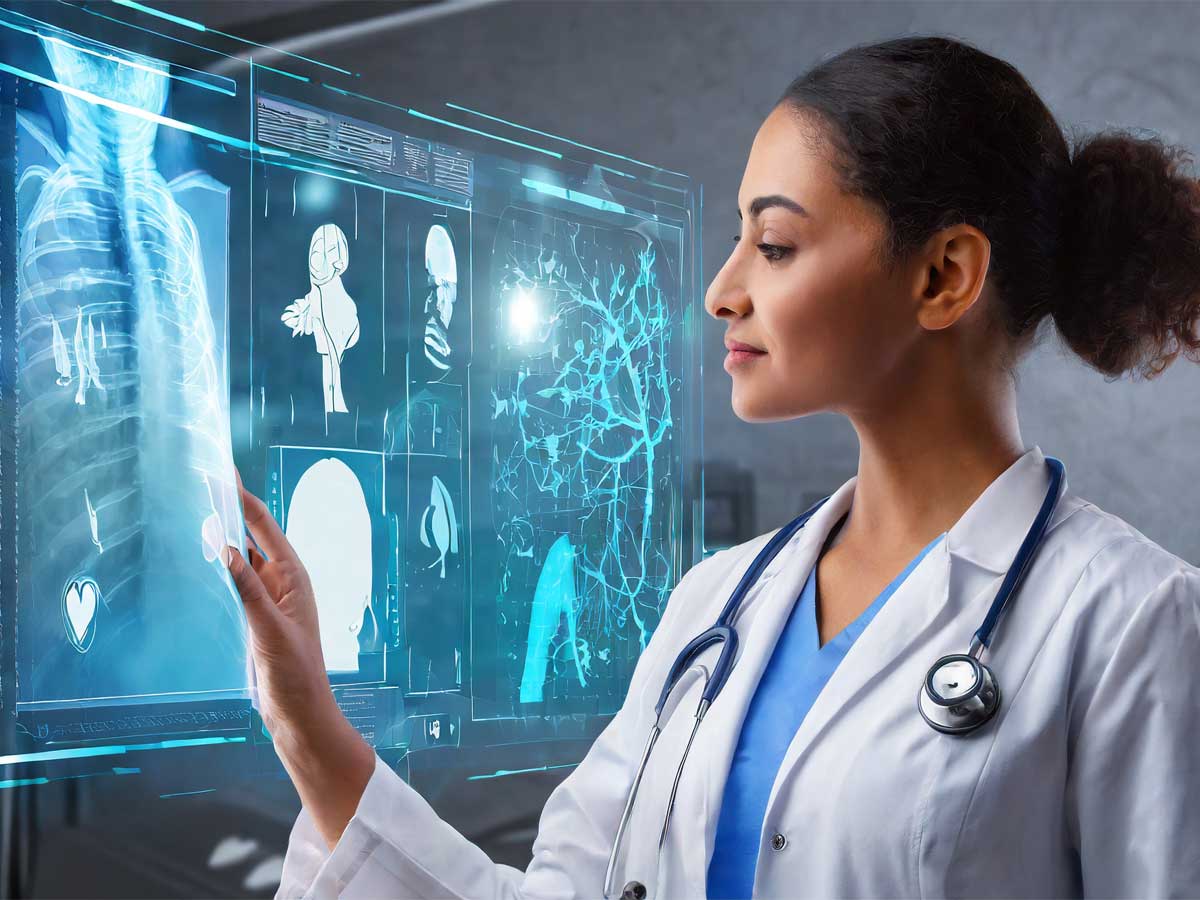
AI also alerts providers to health changes that need attention, ensuring continuous care.
Providing contextual relevance: This is a major advantage of deep learning in medicine. AI algorithms use context to distinguish between different types of information.
For instance, when a clinical note includes a patient's current medications and a new medication recommendation, a well-trained AI algorithm with natural language processing can identify potential conflicts or issues based on the patient's medical history.
Regulatory Implications and Algorithm Limitations
Artificial intelligence algorithms in medicine are showing great promise for both doctors and patients, but they're regulatory roadblocks, and as I always say, if there is AI involved, there will be some gray areas as well, especially in this highly sensitive and important field where privacy and data protection matter. Let me explain this a bit more.
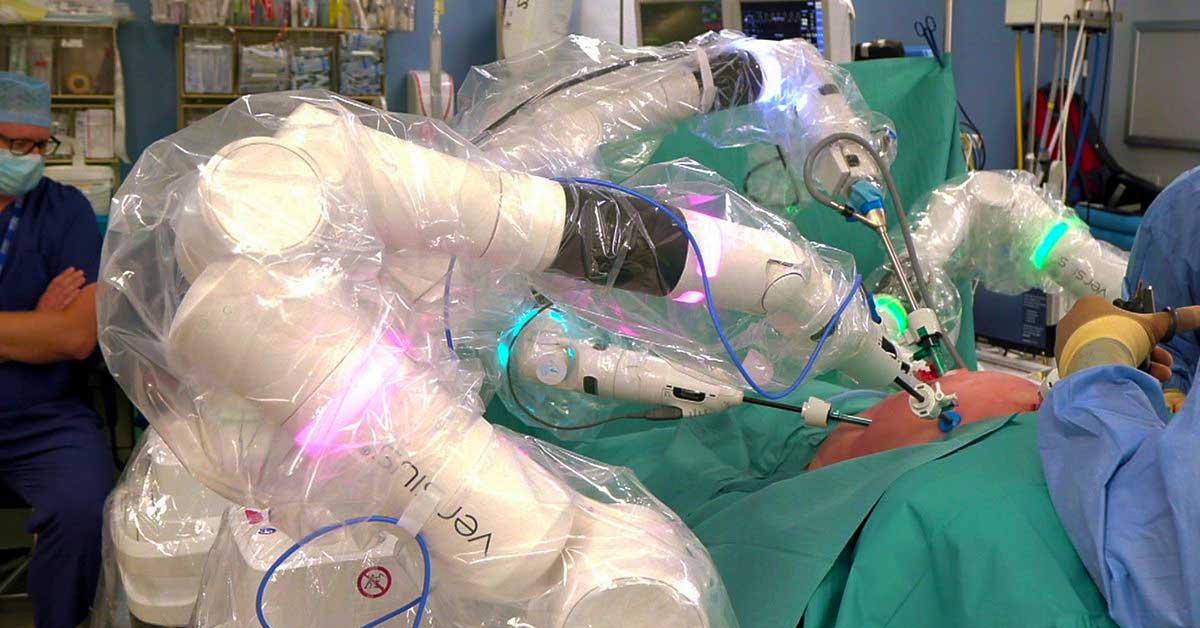
The U.S. Food and Drug Administration (FDA) has given permission, or we can say thumbs up, to some of these smart tools, but there's no one-size-fits-all rulebook for approving them yet.
Basically, there aren't many regulations around AI at all. The people behind these algorithms aren't always the ones in white coats; this means computationalists and doctors need to team up and learn from each other.
While AI is an expert at diagnosing and handling basic clinic stuff and administrative stuff, it's not quite ready for complex tasks like surgeries that need quick, on-the-spot decisions.
The potential of AI in medicine is huge, but it's kind of in a holding pattern, waiting for the FDA to set out clearer guidelines. And the FDA wants total clarity on how these algorithms work, especially those with complex, 'black box' math.
This transparency is a big deal – it could make or break an algorithm's chance of getting the green light for clinical trials. There are already some clinics running trials on these intelligence systems, like the Mayo Clinic Testing Google's Medical AI Chatbot Med-PaLM 2 in Hospitals.
There's also a bit of a difficult situation with keeping algorithm secrets under wraps. On the one hand, you don't want to give away your trade secrets and lose out on patents or get scooped by the competition.
On the other hand, being open about how your algorithm works or making suggestions and decisions is crucial to make sure it's not messing up patient datasets or making wrong calls.
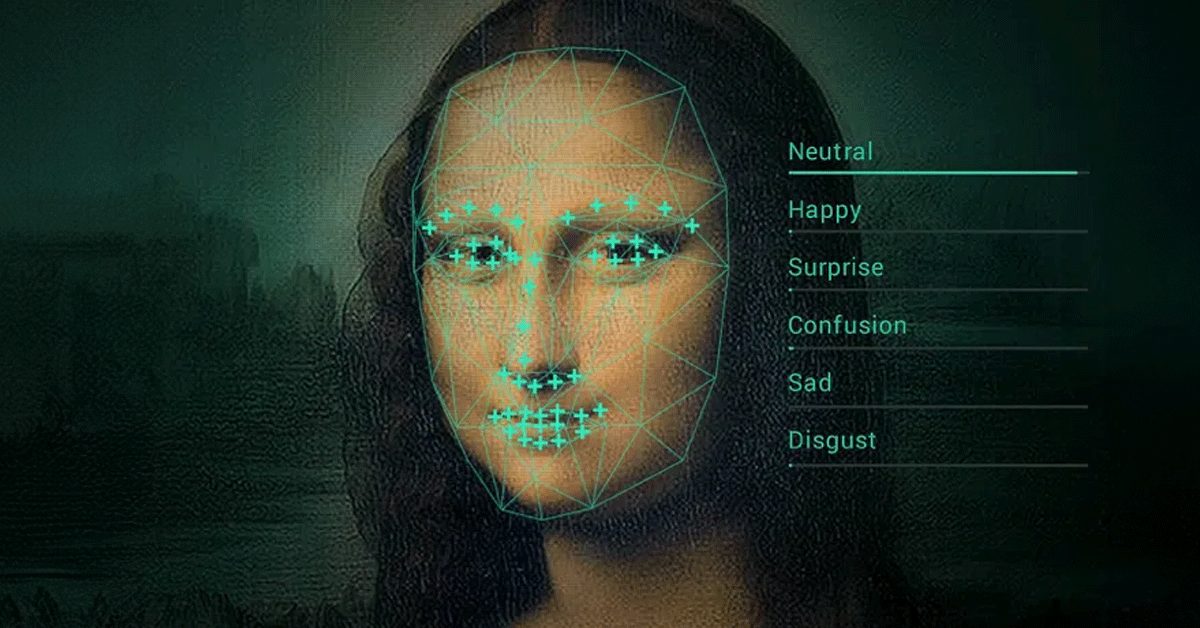
Getting patients to trust these AI algorithms is another hurdle. If people don't get how these programs make their decisions, they might be skeptical about letting it play a role in their healthcare.
There as solutions like Emotion AI, and Ethical AI but just that might not work in these situations. Of course, it's a trick, and people have the right to be skeptical about it. We are talking about their lives.
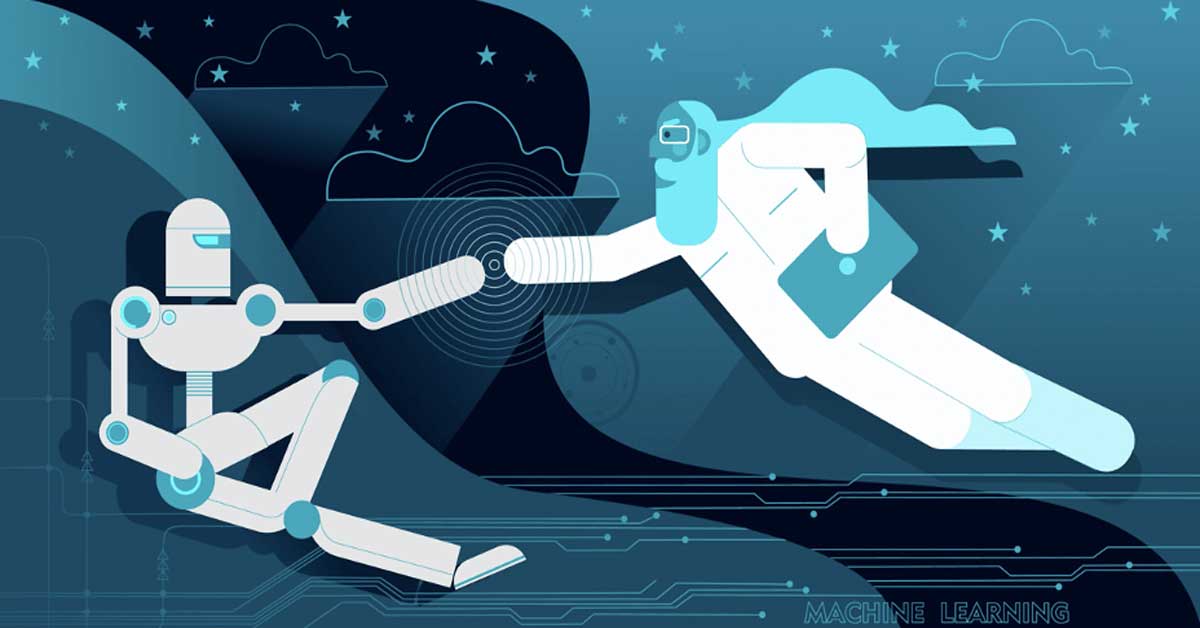
If the data fed into these systems is off, the results will be too. That's why it's super important for the people behind these system developments and the medical pros to work together closely. They need to make sure the algorithms are learning the right things and not going off track.