Ever wondered why your Google Assistant or Siri can't engage in deep conversations about life? That's because they fall under the category of Artificial Narrow Intelligence (ANI).
The term looks fancy, right? I'll explain it in simpler terms, but to go there, we have to start from the beginning.
What is Artificial Intelligence (AI)?
Artificial intelligence is a machine's ability to mimic how the human brain works and intelligence. In some places, it is called machine intelligence. We can divide this into three types of AI:
- Artificial narrow intelligence (ANI), which has a narrow range of abilities and designed to perform one thing;
- Artificial general intelligence (AGI), which is on par with human capabilities or
- Artificial superintelligence (ASI), which is more capable than a human.
Today, we are focusing on one type of AI: Narrow AI.
What is Deep Learning?
The easiest way to explain Deep Learning is that it is one of the more alluring branches of machine learning and explores artificial neural networks with stunning precision to simulate how human minds work.
At its heart, deep Learning relies on data collection as its driving force to enable computers to understand large volumes of information efficiently and quickly.
Deep Learning's hallmark lies in its mysterious depths, hidden layers that fine-tune precision while unlocking automation potential for today's AI innovations.
Consider that everyday items like digital assistants and autonomous vehicles rely heavily on deep Learning's prowess for success. What sets deep Learning apart from conventional machine learning, you ask?
Well, deep Learning excels at handling both structured and unstructured data sources efficiently, extracting features seamlessly without manual manipulation of information!
It takes the burden out of manual data handling by being an efficient data processing platform that handles everything automatically for us. Deep Learning can give your data analysis magic!
Deep Learning encompasses various learning styles: supervised, unsupervised, and reinforcement learning all offer unique capabilities for processing information.
Backpropagation and forward propagation processes combine in an intricate ballet, honing deeper learning into an intimidating but effective weapon.
Real-world applications of deep learning span across sectors like law enforcement, financial services, customer service, and healthcare, taking on roles like speech recognition systems or medical image analysis as examples of its magic in action.
Deep learning hardware investments should also be expected; remember this when witnessing jaw-dropping speech recognition or medical image analysis systems at work!
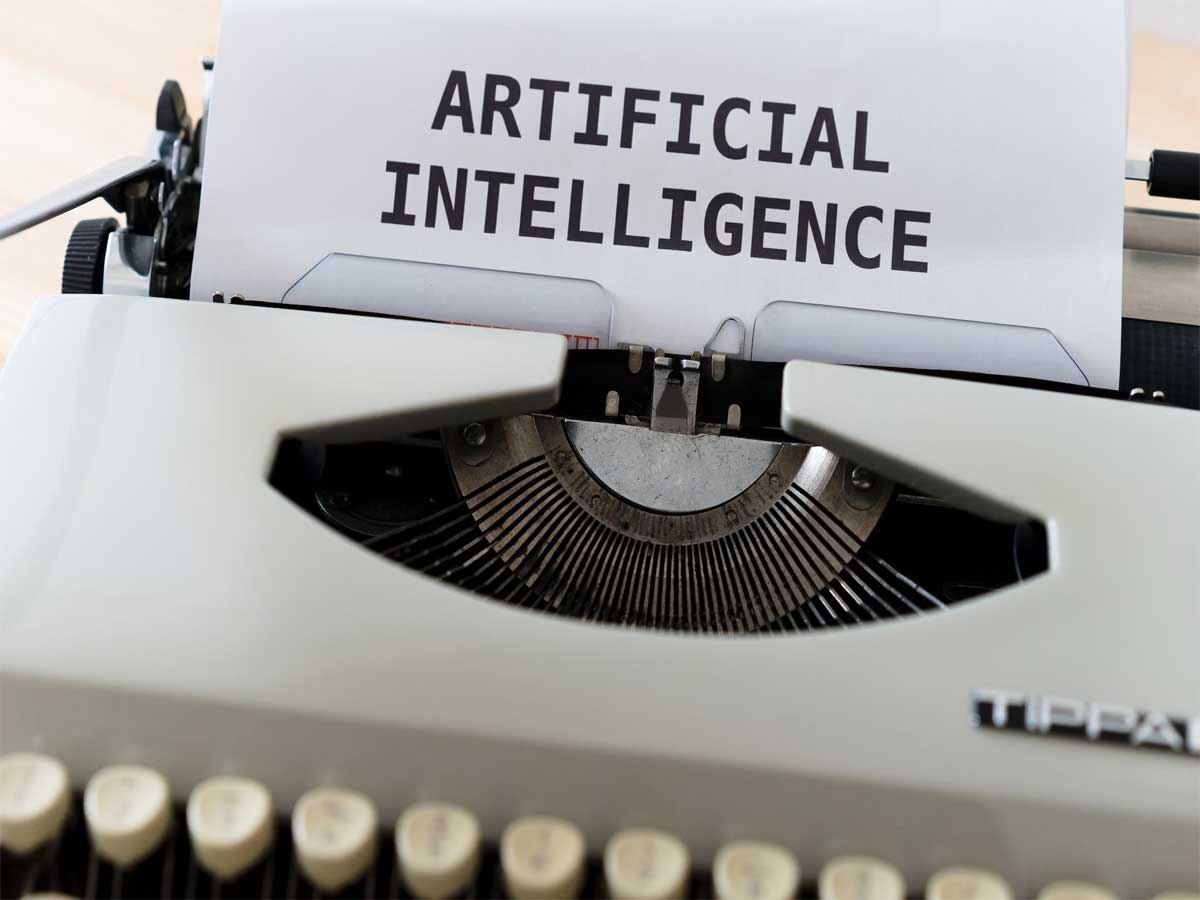
What is Narrow AI / Weak AI?
Narrow AI, also known as Weak AI or Artificial Narrow Intelligence, is an Artificial Intelligence (AI) system designed to replicate and perhaps surpass human intelligence for a dedicated purpose to perform a specific task or a set of closely related tasks.
They're programmed to pull info from a specific dataset and stick to the task at hand, unlike the hypothetical Artificial General Intelligence (AGI), also known as Strong AI, which mimics human smarts across the board. It's basically a theoretical AI system that could be applied to any task or problem.
What can Narrow AI do?
The powers of narrow artificial intelligence are pretty remarkable within their specific territory. These systems are great at accurately analyzing massive datasets, spotting patterns and trends, and making smart predictions or decisions based on the data.
They can also get better over time, thanks to techniques like machine learning and deep learning.
Yet, there's a catch. Narrow AI cant do abstract thinking, understanding context beyond its programmed scope, or flaunting emotional smarts. Their learning is confined to the rules set by their algorithms and the data they've been trained on.
What is a Neural Network?
When we discuss about deep learning, we have to mention neural networks. Basically, a neural network is a technique used in the field of advanced artificial intelligence that aims to replicate the way the human brain processes information, and it's an important part of deep learning; these networks are structured with interconnected nodes or neurons, much like the neurons in our brains.
They come in handy for tasks such as recognizing handwriting and identifying faces. On the other hand, artificial intelligence (AI) seeks to mimic human cognitive abilities.
These Neural Networks draw inspiration from biological neural networks, forming a bridge between the natural and artificial.
Learning within these networks involves adjusting the weights of connections, a process categorized into three main types: supervised learning, unsupervised learning, and reinforcement learning.
There are nine different types of neural networks, each with its unique characteristics. These include the Perceptron, Forward Neural Network, Multilayer Perceptron, Convolutional Neural Network, Radial Basis Functional Neural Network, Recurrent Neural Network, LSTM – Long Short-Term Memory, Sequence Sequence Models, and Modular Neural Network.
Examples of Narrow AI In real life
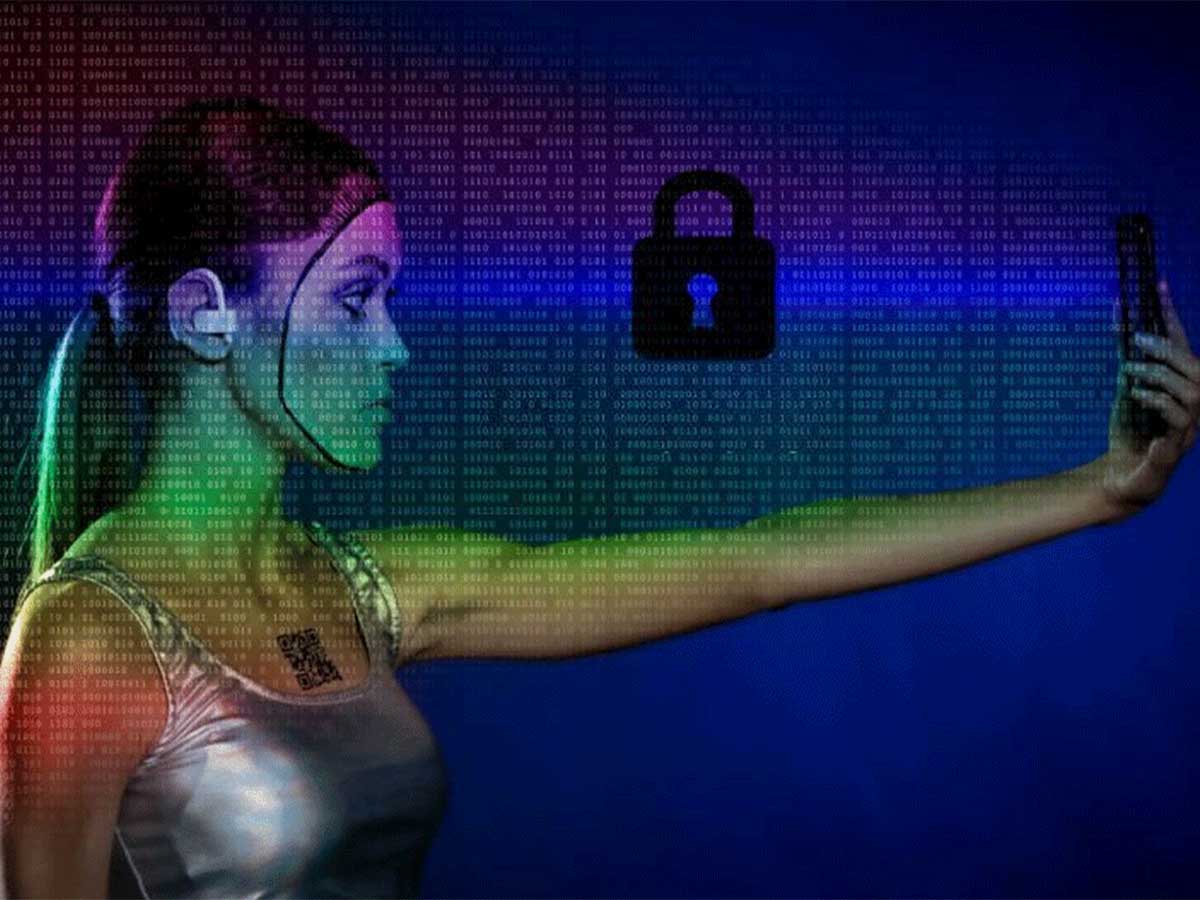
Narrow AI is being used in most of the files that AI is already enhancing. Basically, modern AI systems are mostly in this category.
Image and Facial Recognition Systems:
Social media giants like Facebook and Google use these systems to automatically identify individuals in photographs, representing a form of weak AI.
Chatbots and Conversational Assistants:
Inclusive of popular virtual assistants like Google Assistant, Siri, and Alexa, as well as simpler customer-service chatbots, these applications demonstrate the capabilities of narrow AI in human-computer interactions.
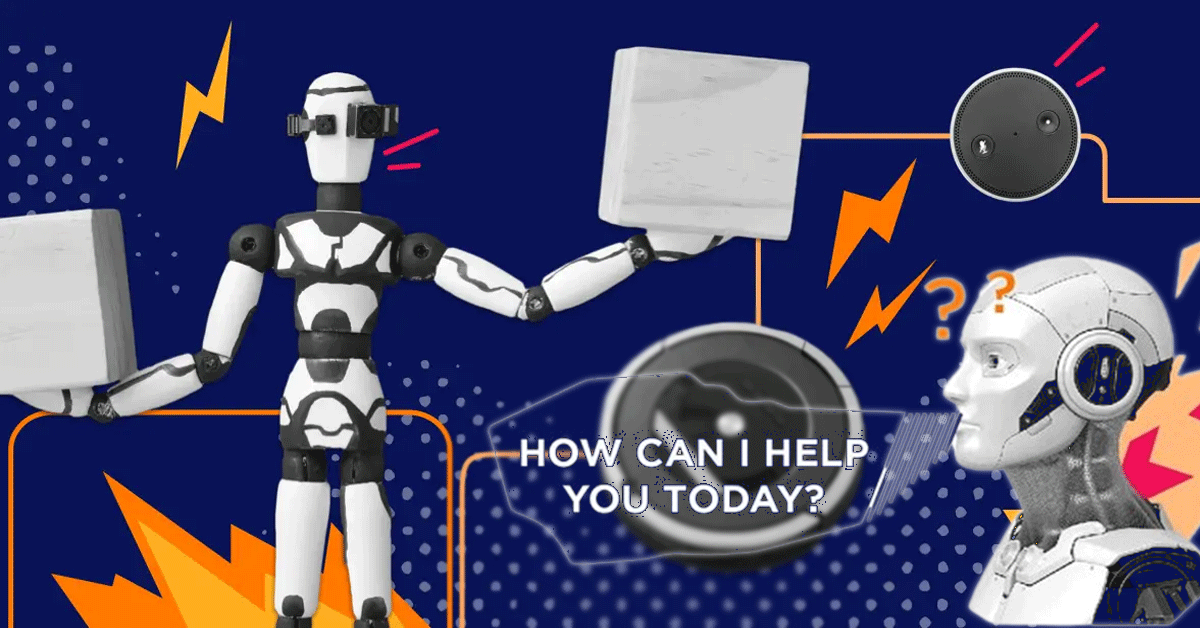
Self-Driving Vehicles:
Seen in autonomous or semiautonomous cars, including certain Tesla models, as well as autonomous drones, boats, and factory robots, narrow AI contributes to the development of intelligent machines in the transportation and manufacturing sectors.
Predictive Maintenance Models:
Drawing insights from data collected through sensors, these models play a crucial role in predicting potential machine failures offering timely alerts and preventive measures.
Recommendation Engines:
Powering platforms that predict user preferences or next search queries, such as those seen in content streaming services recommendation engines.
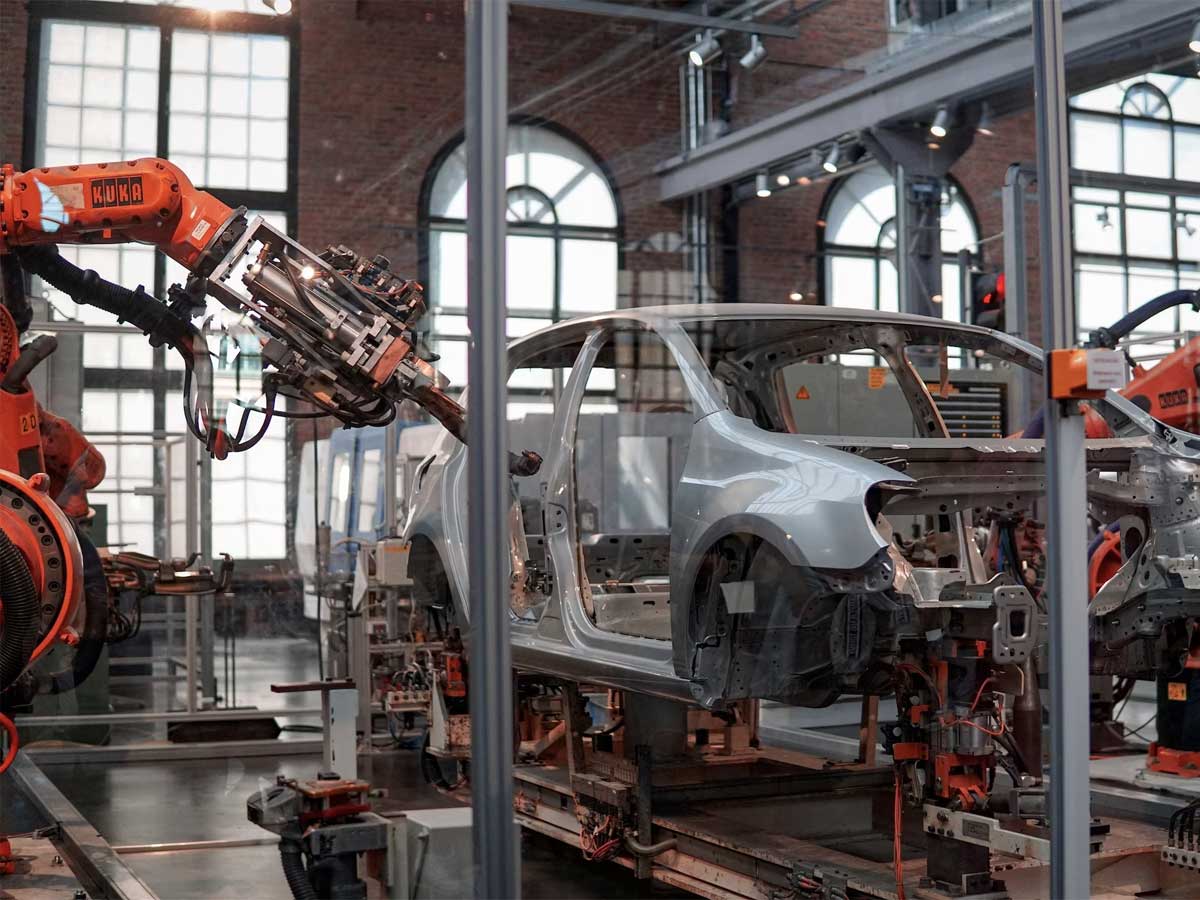
Healthcare:
In the medical field, AI algorithms support disease diagnosis, analyze medical images, and predict patient outcomes, highlighting the valuable role of narrow AI in healthcare applications.
Finance:
Widely applied in finance, AI contributes to algorithmic trading, fraud detection, and customer service automation within the banking sector, showcasing its impact on efficiency and security.
Manufacturing:
Within manufacturing processes, AI optimization improves efficiency, supply chain management, and quality control, demonstrating its role in enhancing productivity and product quality.
Language Translation Services:
Platforms like Google Translate leverage narrow AI to provide accurate and context-aware translations, breaking language barriers on a global scale and showcasing the practical applications of AI in language services.
What is Machine learning (ML)?
Machine learning is basically a branch of artificial intelligence.
Artificial intelligence is a machine's ability to mimic how the human brain works and intelligence. Machine learning is a part of AI that uses algorithms to teach computers to learn and improve their processes.
Machine learning algorithms have the ability to enhance their performance over time as they are trained with large amounts of data. These algorithms generate machine learning models that contain the knowledge gained from running algorithms on training data.
The more data that is used to train the algorithm, the more accurate and refined the model will become.
natural language processing
In natural language processing, machine learning models empower virtual assistants such as Alexa, Google Assistant, and Siri to comprehend and respond to human language.
Advantages and Challenges of Narrow AI
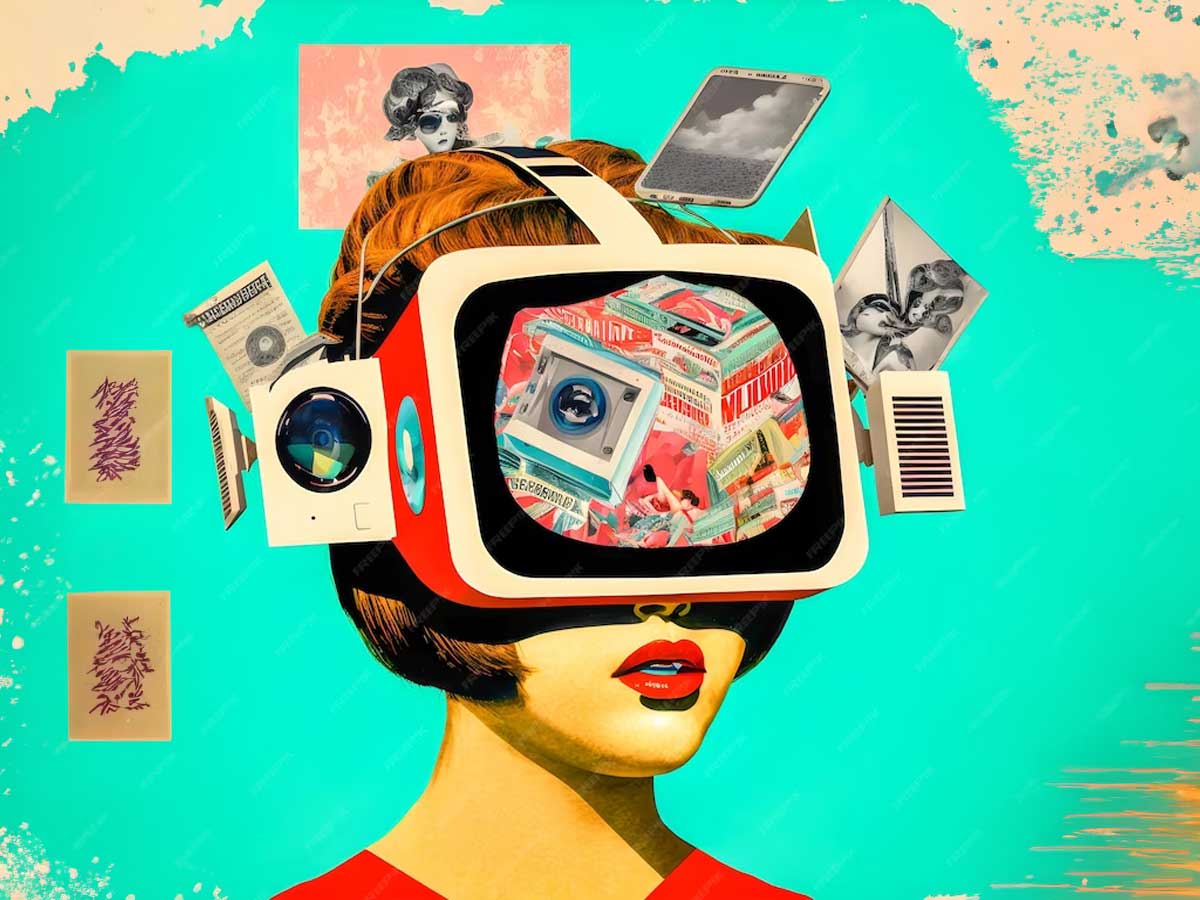
What are the Benefits of Narrow AI?
Facilitating Faster Decision Making:
Artificial narrow intelligence systems expedite decision-making by swiftly processing data and completing tasks at a pace surpassing human capabilities. AI helps us to enhance overall productivity and efficiency, improving the quality of life.
Consider IBM's Watson, an example of such a narrow AI system, which aids doctors in making data-driven decisions.
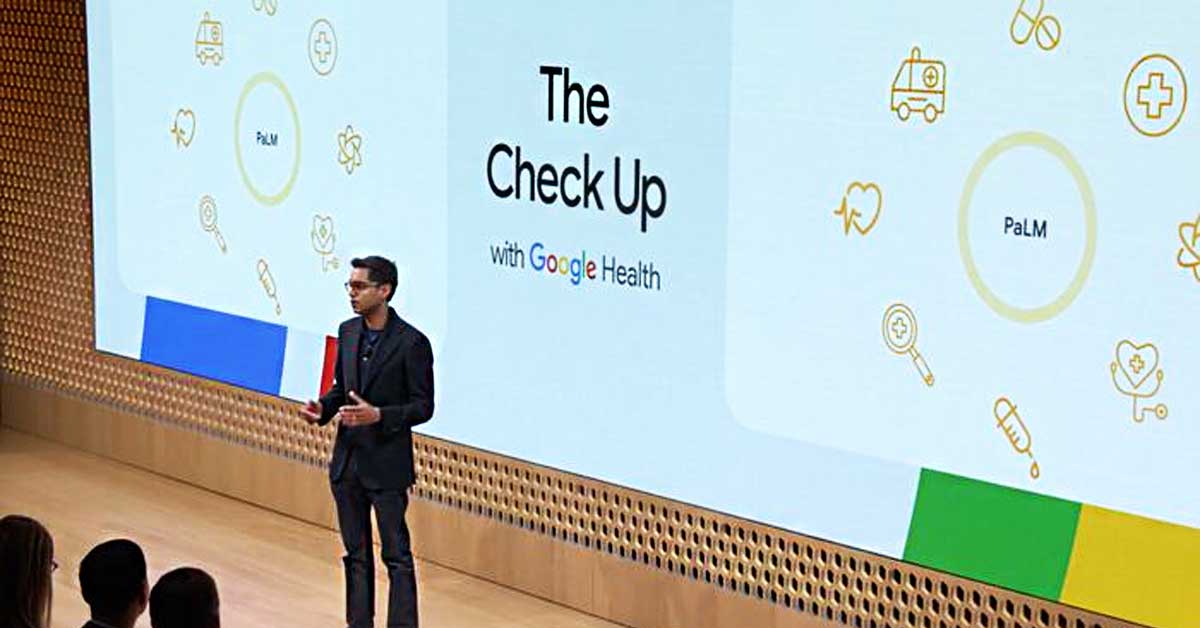
Relieving Humans from Mundane Tasks:
Advancements in narrow AI have guaranteed that individuals are freed from numerous boring and mundane tasks. This has simplified our daily routines, from utilizing Siri to ordering food online to streamlining extensive data analysis for quicker results.
Furthermore, innovations like self-driving cars have alleviated the stress and hassle of prolonged traffic jams, offering us more leisure time to engage in activities or pursuits of personal interest.
Serving as a Building Block for More Intelligent AI:
Artificial narrow intelligence systems play a key role in AI, acting as the groundwork for the evolution of more sophisticated versions like general AI and super AI—speech recognition, where computers skillfully convert spoken words into text with remarkable precision. Meanwhile, computer vision assigns systems to recognize and categorize objects seamlessly within video streams.
Performing Single Tasks Better Than Humans:
Narrow AI excels at specific tasks, sometimes even outshining humans. Need a cancer diagnosis from X-ray images? Let the AI system handle it; it might beat a radiologist at the speed and accuracy game. Predictive maintenance systems? They analyze sensor data in real-time.
Availability of Narrow AI:
These systems are the 24/7 workers of the digital world. They adapt to the workload, scaling up or down as needed.
Reduction of Human Error:
By automating tasks, it helps cut down on those human errors that can sometimes have serious consequences.
Improved Safety with Narrow AI:
In certain industries like manufacturing and transportation, safety is paramount. Narrow AI takes on risky tasks, making workplaces safer for humans.
What is Artificial Superintelligence (ASI)?
Imagine a world where machines go beyond just being smart; we're talking about Artificial Superintelligence (ASI) or, as it's coolly known, Super AI, a hypothetical AI that doesn't just copy human smarts but becomes self-aware and outsmarts us entirely.
Think of the scenes in those dystopian sci-fi movies where robots go wild, overrun, overthrow, and/or enslave humanity – that's the kind of drama ASI brings. The idea is for AI to evolve to understand not just our intelligence but our feelings, too, evoking emotions, needs, beliefs, and desires of its own.
The unknown consequences of having super-smart, self-aware beings include the possibility of them having ideas like self-preservation. The impact on humanity, our survival, and our way of life is still in pure speculation.
What are the Limitations of Narrow AI?
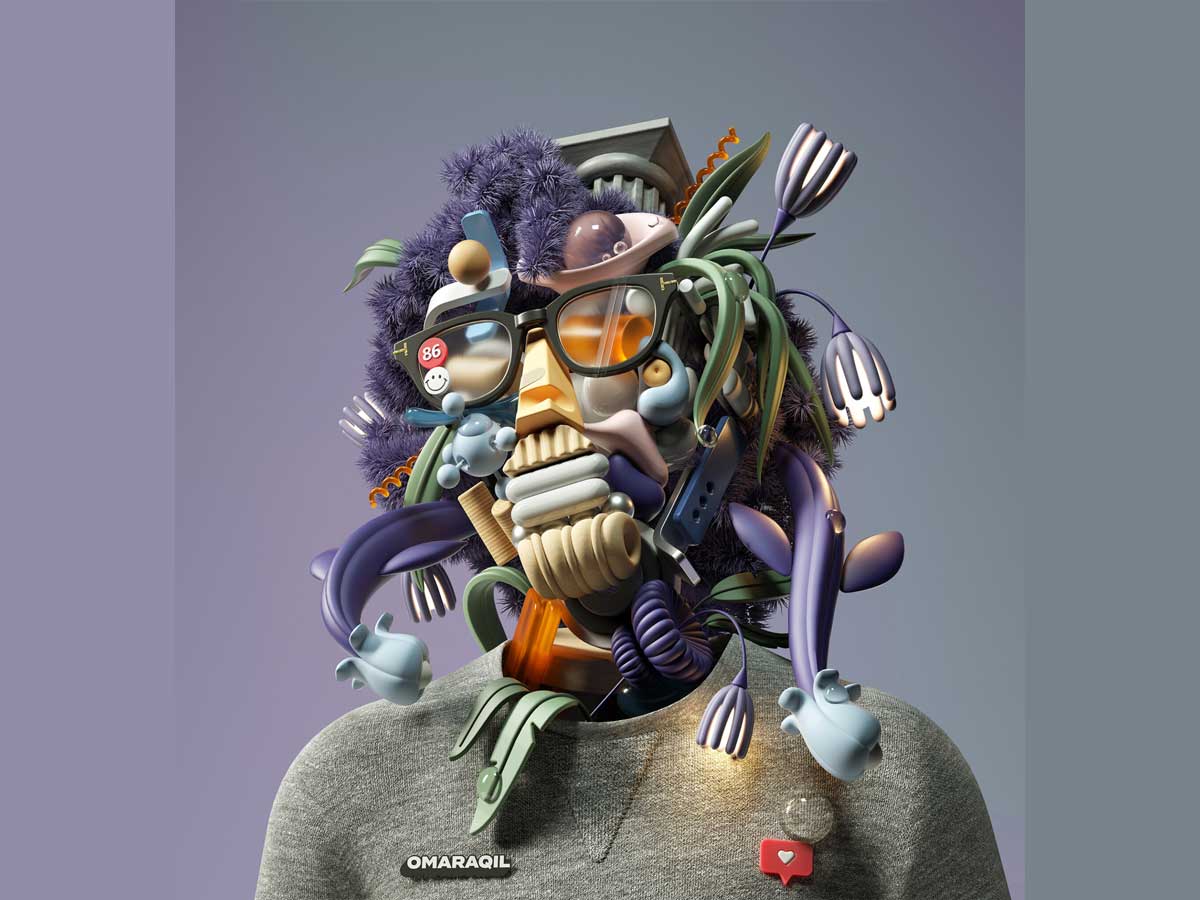
Narrow AI does have its limitations, too. So, let's look into that.
Lack of Flexibility:
Narrow AI systems are specialists; they're designed for specific gigs and won't budge an inch beyond that.
Dependency on Data:
These AI champs are data-dependent. Their performance relies heavily on the data they've been trained on.
Lack of Understanding:
Don't be fooled by the apparent smarts – Narrow AI doesn't truly understand what it's doing. It follows pre-programmed instructions without grasping the deeper context. Medical AI might spot patterns in images but lacks the understanding of the biological intricacies behind them.
Absence of Explainable AI:
The mystery box problem. Current AI operates like a magician's hat, making decisions without revealing the tricks in between. This is all fun and games until it's making crucial business decisions. The challenge is to make AI more transparent and less of a black box.
Need for Impenetrable Security:
Security is a chink in the armor. Neural networks in Narrow AI are susceptible to attacks. Hacking into the software of autonomous cars to mess with how AI perceives the world? That's a real concern.
Need to Learn from Small Data:
It's a big data world, but AI needs to be minimalist. Teaching AI to learn more from less data and transfer knowledge between networks is the goal.
Prone to Bias:
Bias creeps in. If the training data has biases, AI inherits them. Picture a credit decision system echoing outdated biases, impacting fair lending. Ensuring AI training data is bias-free is a real challenge. Because every person is biased toward some sort of belief.
Top 10 Best Practices for Narrow AI Development:
Here are the top 10 best practices for narrow AI development.
Human-centric design approach:
- Augmentation and assistance: AI should be your helpful sidekick, not a know-it-all. Let it suggest options and give diverse answers that cater to everyone.
- Potential adverse feedback: Think of AI as a friend needing constructive criticism. Incorporate feedback during its design, testing, and growth.
- A diverse set of users and use-case scenarios: AI should be a friend to all. Involve diverse users and think about all the different situations they might encounter.
Metrics to assess training and monitoring:
- Essential metrics: Think of metrics as the AI report card. Check user surveys, system performance, and tricky false positives/negatives.
- Context and goals: Make sure the report card makes sense. Align metrics with what the AI is meant to do.
Periodic examination of raw data:
- Analyzing raw data: Before you let AI loose, check its homework. Look for missing stuff and wrong labels, and make sure it's not picking favorites.
- Addressing data bias: No one likes a biased friend. Analyze raw data to make sure AI isn't playing favorites.
Consider the limitations of the AI dataset and model:
- Correlation detecting AI model: AI might get correlations wrong, so don't jump to conclusions.
- Scope and coverage of training: Like a GPS, be clear about where AI has been trained and where it might not work well.
- Communicate limitations: Tell users where AI might goof up.
Ensure the AI system works as intended by performing tests:
- Unit tests: Check each part separately, like making sure the wheels on a car don't have a mind of their own.
- Integration tests: Make sure all parts play nice together.
- Iterative user tests: Ask users what they think.
- Quality checks: Build safety nets.
Regularly monitor and update the AI system post-deployment:
- Clear roadmap: Have a plan for fixing stuff.
- Balancing short- and long-term fixes: Fix things for now and for the future.
- Understanding update impact: Check before you hit "update."
Ensure fairness:
- Impact analysis: See how AI affects different folks.
- Define goals: Set goals for everyone, not just a select few.
- Objective function and algorithms: Make sure AI plays fair.
- Regular monitoring: Keep an eye out for unfair moves.
Consider interpretability:
- Collaboration with domain experts: Get help from the experts.
- Post-training interpretability options: Figure out how to understand AI's secrets.
- Analysis of training or testing data: See what's under the hood.
- Transparency balance: Make sure AI isn't too mysterious.
Ensure privacy:
- Tests using metrics: Check if AI accidentally exposes secrets.
- Experimentation with variables: Play with the settings to keep things private.
Provide security:
- Rigorous threat model: Imagine all the ways AI could get into trouble.
- Identification of unintended consequences: Look out for surprises.
- Development of methods: Have tricks up your sleeve.
- Performance testing in hostile settings: See how well AI handles tough situations.
Unlike its ambitious counterpart, general AI, which remains more aspirational, narrow AI systems are the workhorses that surround us today. They are specialized and efficient and perform specific tasks like conversing with Siri or captioning millions of images.
The benefits of narrow AI are evident in its applications across various domains, outperforming humans in tasks and ensuring that AI systems are both practical and powerful.