In today's world, where smartphones rule, taking amazing photos has become crucial to our everyday routines. But have you ever wondered how some smartphones produce jaw-dropping images that rival professional cameras?
There is a secret, and it lies not only in cutting-edge hardware but also in the power of software algorithms. The Google Pixel is one of the smartphones leading the revolution in Mobile Photography.
How does the Google Pixel camera defy hardware limitations to deliver exceptional photography experiences?
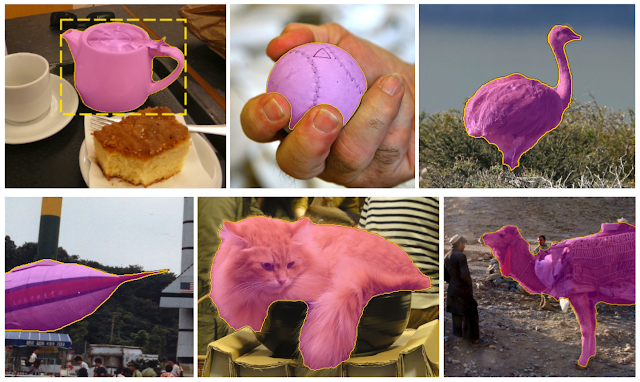
Let me tell you about the incredible capabilities of the Pixel camera, which relies on complex software algorithms rather than solely on hardware advancements.
Get ready to explore the fascinating world of computational photography as we unravel the secrets behind the Pixel camera's extraordinary performance.
The Pixel Camera: A Software-Driven Marvel
The Google Pixel camera stands apart from its competitors by harnessing the power of software algorithms, pushing the boundaries of what can be achieved with smartphone photography. Let's explore some key features and technologies that make the Pixel camera a true marvel.
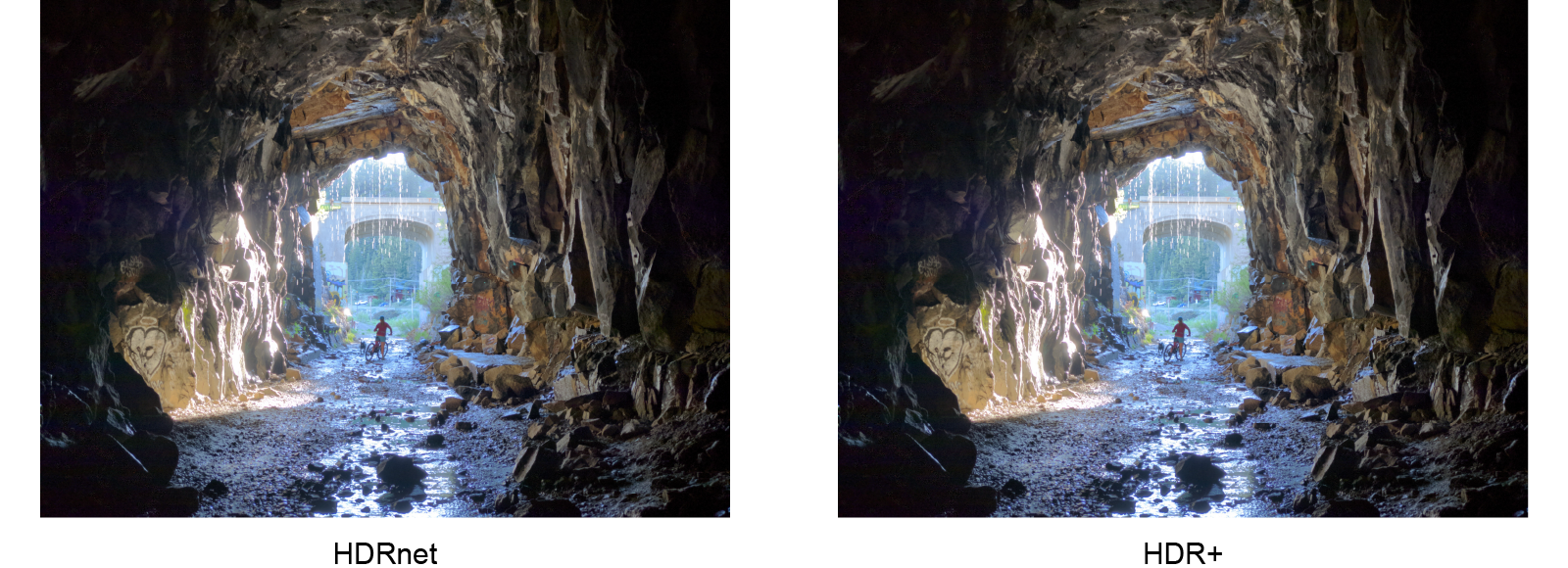
HDR+ Mode: Enhancing Image Quality
One of the standout features of the Pixel camera is its HDR+ mode, which revolutionizes image quality. Unlike traditional smartphone cameras that rely solely on a single exposure, the Pixel camera takes a different approach. It captures multiple underexposed shots of the same scene and combines them with mathematical calculations.
By capturing multiple shots with different exposure levels, the Pixel camera can gather a wealth of information and detail from both the highlights and shadows. These shots are then intelligently merged, leveraging the power of computational photography. The result? An image with balanced exposure, reduced noise, and remarkable dynamic range.
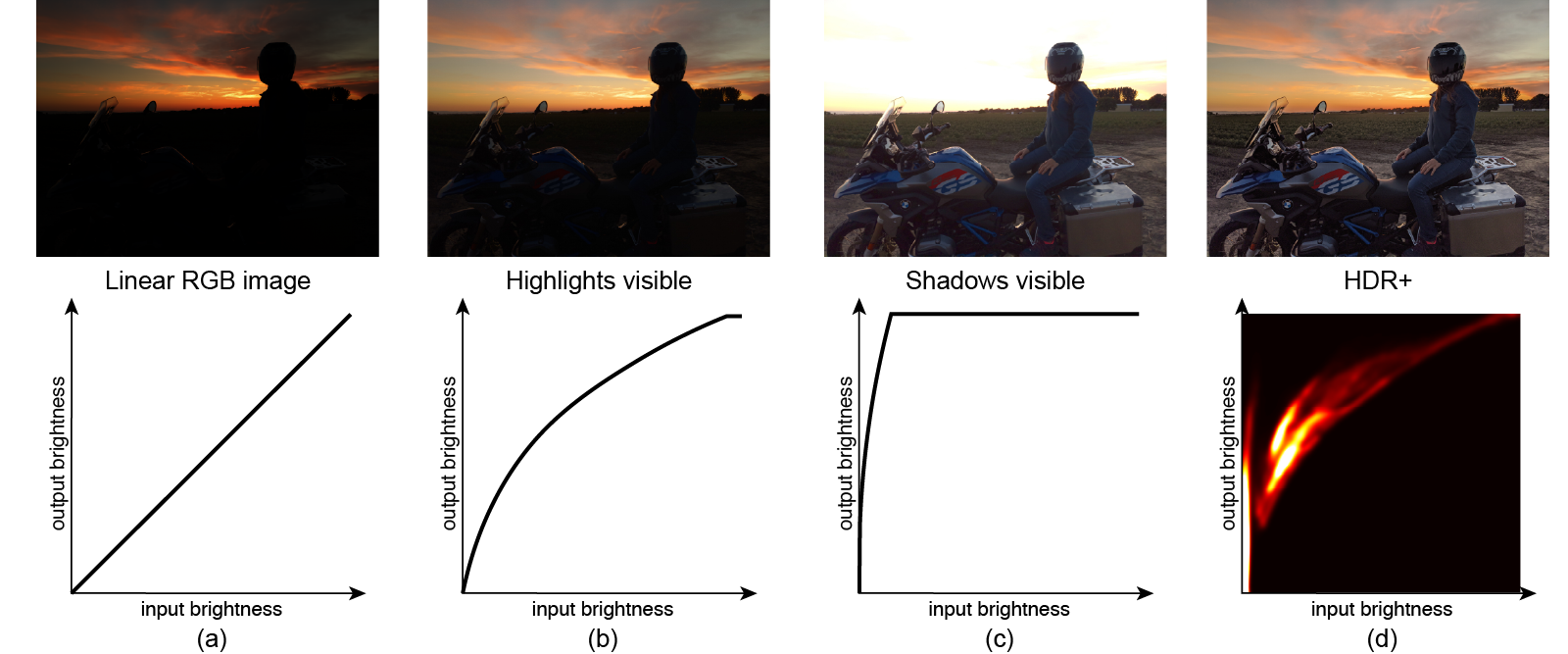
Moreover, HDR+ mode works wonders in low-light situations, where capturing details can be challenging. By intentionally underexposing each shot, the Pixel camera improves its low-light performance and preserves color saturation, even in dimly lit environments. The result is vibrant, well-lit photos that capture the moment's essence.
The Hexagon Digital Signal Processor: Zero Shutter Lag
To ensure an exceptional photography experience, the Pixel camera is equipped with a Hexagon digital signal processor. This advanced technology plays a crucial role in delivering zero shutter lag, allowing users to capture images instantaneously without any delay.
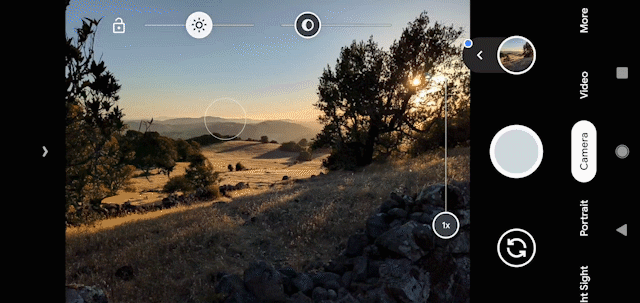
The Hexagon DSP enables the Pixel camera to capture RAW imagery, which means it can retain the original, unprocessed data captured by the camera sensor. This capability gives users greater control and flexibility in post-processing, as RAW images contain more information and offer more room for adjustments compared to compressed image formats.
Advancing Camera Technology: Google's Vision
Google's vision for the future of smartphone photography goes beyond hardware advancements. They strive for more vertical integration in camera technology and explore the vast possibilities of software-defined computational photography.
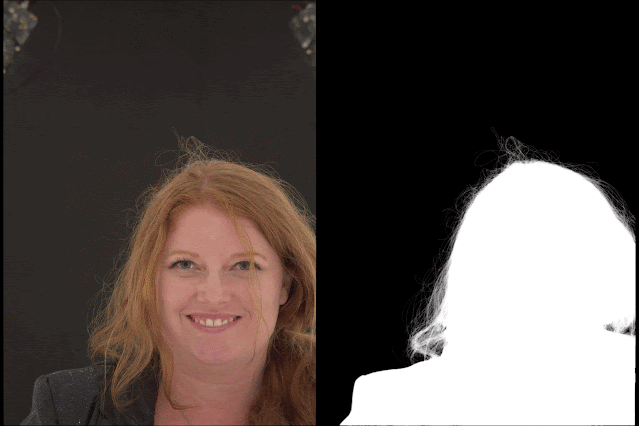
By embracing software-driven solutions, Google aims to enhance the capabilities of the Pixel camera even further.
They continuously develop advanced algorithms to improve image quality, optimize processing, and unlock new creative features. This approach allows them to iterate and innovate rapidly, delivering cutting-edge imaging capabilities to Pixel users through software updates.
Alpha Matting: Precision in Separating Foreground and Background
When it comes to capturing and manipulating images, accurately separating the foreground and background objects is crucial. Traditional image segmentation techniques often need help with fine details like hair and need to provide precise boundaries.
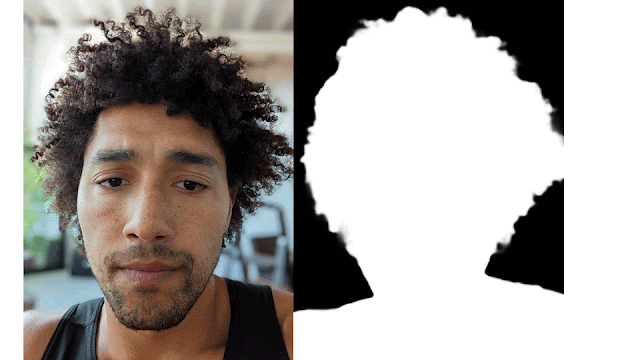
However, alpha matting has revolutionized this process, unlocking a new level of precision and detail.
Traditional Image Segmentation Limitations
Traditional image segmentation methods have their limitations, especially when it comes to intricate details like hair. These techniques often struggle to accurately identify the boundaries between the foreground and the background, resulting in subpar results. The lack of precision can lead to artifacts and inconsistencies in the final image, diminishing the overall quality and realism.
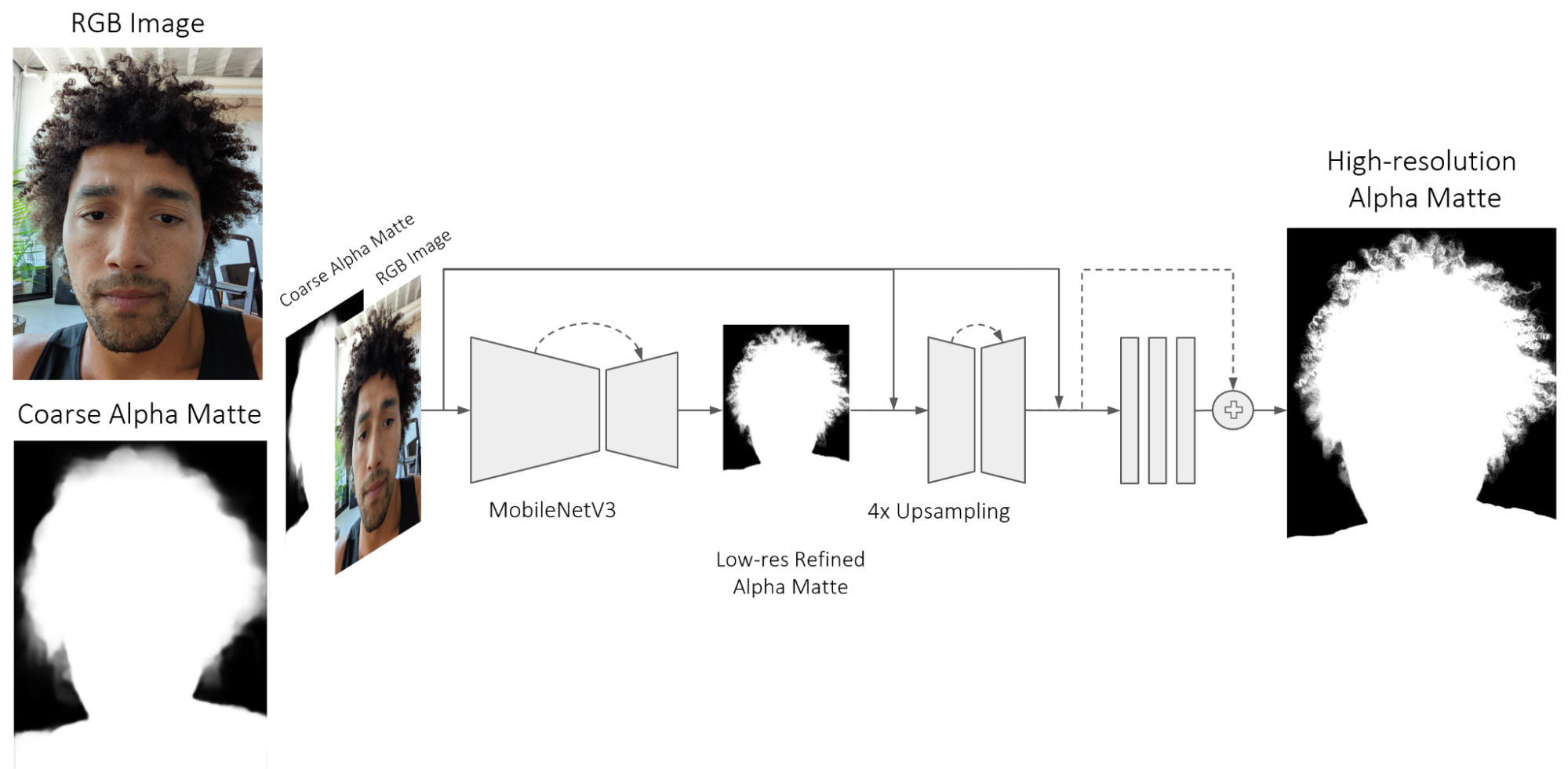
Furthermore, traditional methods fail to capture the subtleties required for accurate foreground-background separation. Subtle elements such as wisps of hair, delicate textures, or intricate patterns often get overlooked or improperly rendered. This limitation hampers the ability to create realistic compositions and manipulate images with the desired level of precision.
Alpha Mattes: Unleashing the Potential
In contrast to traditional image segmentation, alpha mattes provide a powerful solution for precise foreground-background separation. An alpha matte is a grayscale image that acts as a mask, indicating the opacity of each pixel. By accurately estimating and applying alpha mattes, images can be seamlessly composited, extracted, or modified with incredible precision.
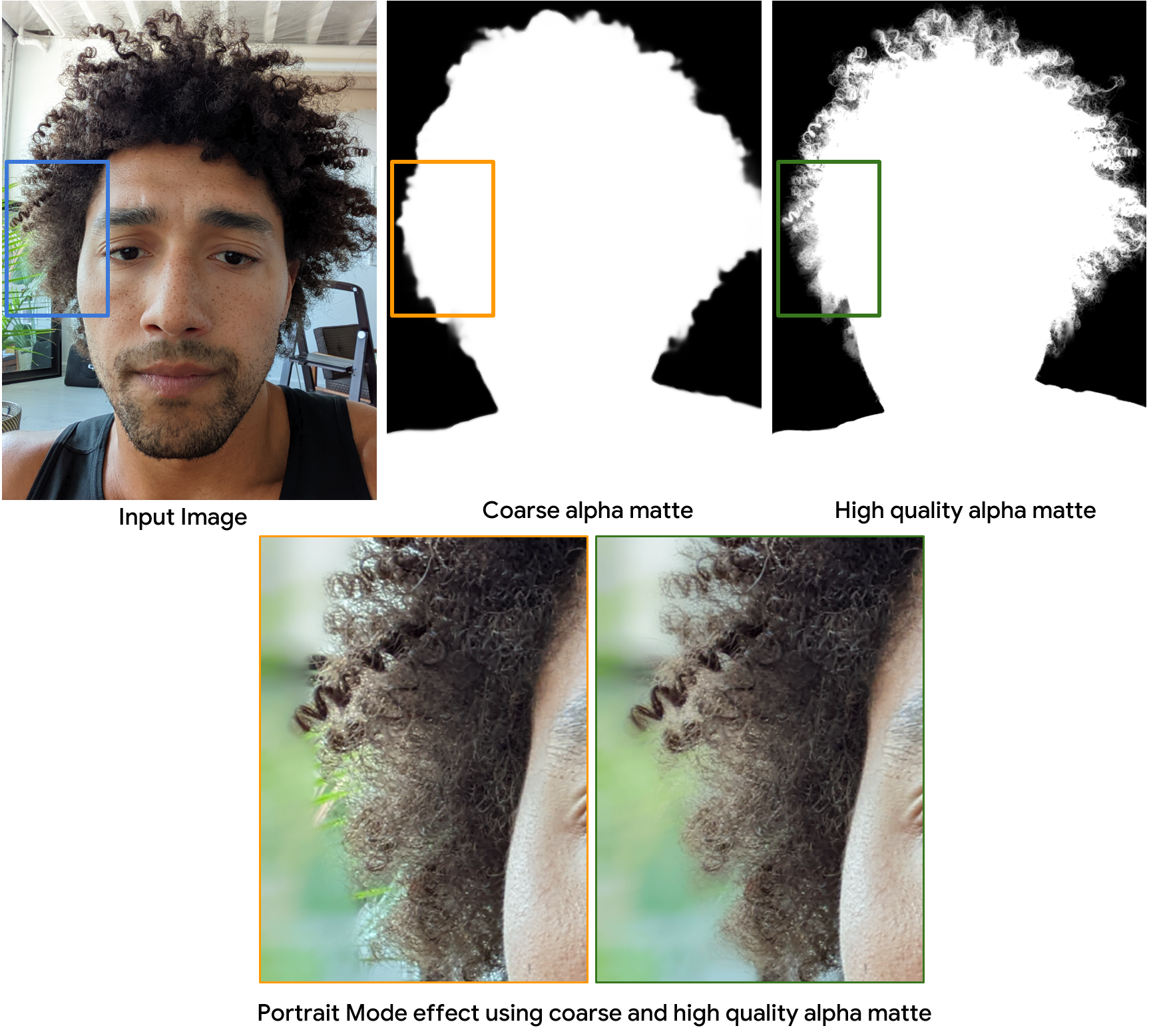
The magic of alpha mattes lies in their ability to preserve intricate details, including subtle elements like hair. Unlike traditional techniques, alpha mattes allow for precise boundaries, resulting in cleaner and more natural compositions. This level of accuracy opens up new possibilities for advanced image editing and manipulation.
With alpha mattes, foreground objects can be seamlessly extracted from their original backgrounds and placed onto new ones, creating stunning visual effects and realistic composites. The ability to handle complex scenes and maintain fine details allows for more creative freedom and enhances the overall quality of the final image.
Deep Learning in Image Matting

Deep learning techniques have shown great potential in improving image matting accuracy. These approaches aim to learn complex mappings between input images and accurate alpha mattes by leveraging neural networks. However, they also face their fair share of challenges.

One of the main challenges in deep learning-based image matting is the need for accurate ground truth data. Training deep learning models requires a large dataset with meticulously annotated alpha mattes. Obtaining such data can be laborious and time-consuming, limiting high-quality training sets' availability.
Another challenge lies in the generalization of deep learning models. The ability of a model to accurately estimate alpha mattes from a wide range of images and scenarios is crucial. Ensuring that the learned representations generalize well to unseen data remains an ongoing area of research and development.
The Evolution of Portrait Mode
In smartphone photography, capturing stunning selfies has become an essential feature. With the release of the Pixel 6, Google has taken portrait mode to new heights, enhancing the quality and realism of selfies like never before. One of the key advancements lies in estimating high-resolution alpha mattes, which play a vital role in separating the subject from the background with exceptional detail and accuracy.
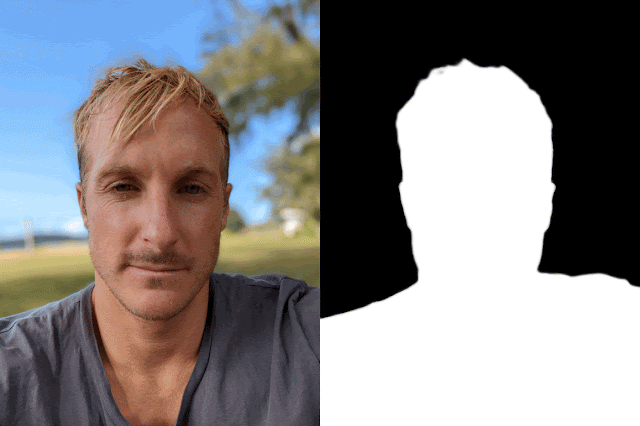
Enhancing Selfies with High-Resolution Alpha Mattes
With the Pixel 6, portrait mode selfies have significantly improved, thanks to the incorporation of high-resolution alpha mattes. These alpha mattes provide precise information about the opacity of each pixel, enabling a clean and accurate separation of the subject from the background. As a result, selfies taken with the Pixel 6 exhibit enhanced depth and realism.
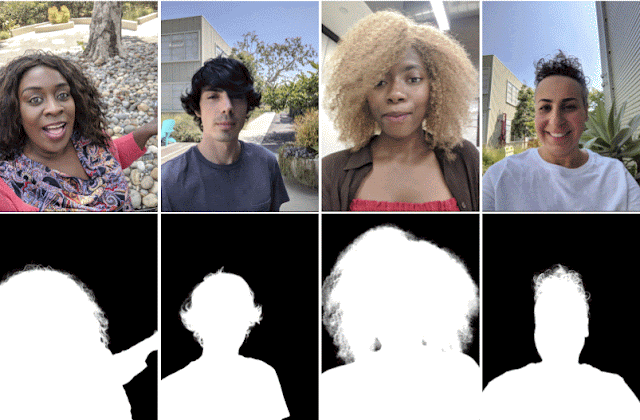
Estimating high-resolution alpha mattes involves capturing and processing an extensive amount of data. By analyzing the intricate details of the subject and its surroundings, the Pixel 6's camera system can generate alpha mattes that preserve the fine contours, edges, and textures, even in complex scenes. This advancement in selfie technology allows for more immersive and lifelike portraits.
Portrait Matting: The Backbone
At the core of the Pixel 6's portrait mode lies a powerful convolutional neural network (CNN) known as Portrait Matting. This CNN is designed to tackle the challenges of accurately estimating alpha mattes for portrait images. It leverages a MobileNetV3 backbone, a state-of-the-art network architecture known for its efficiency and effectiveness in image analysis tasks.
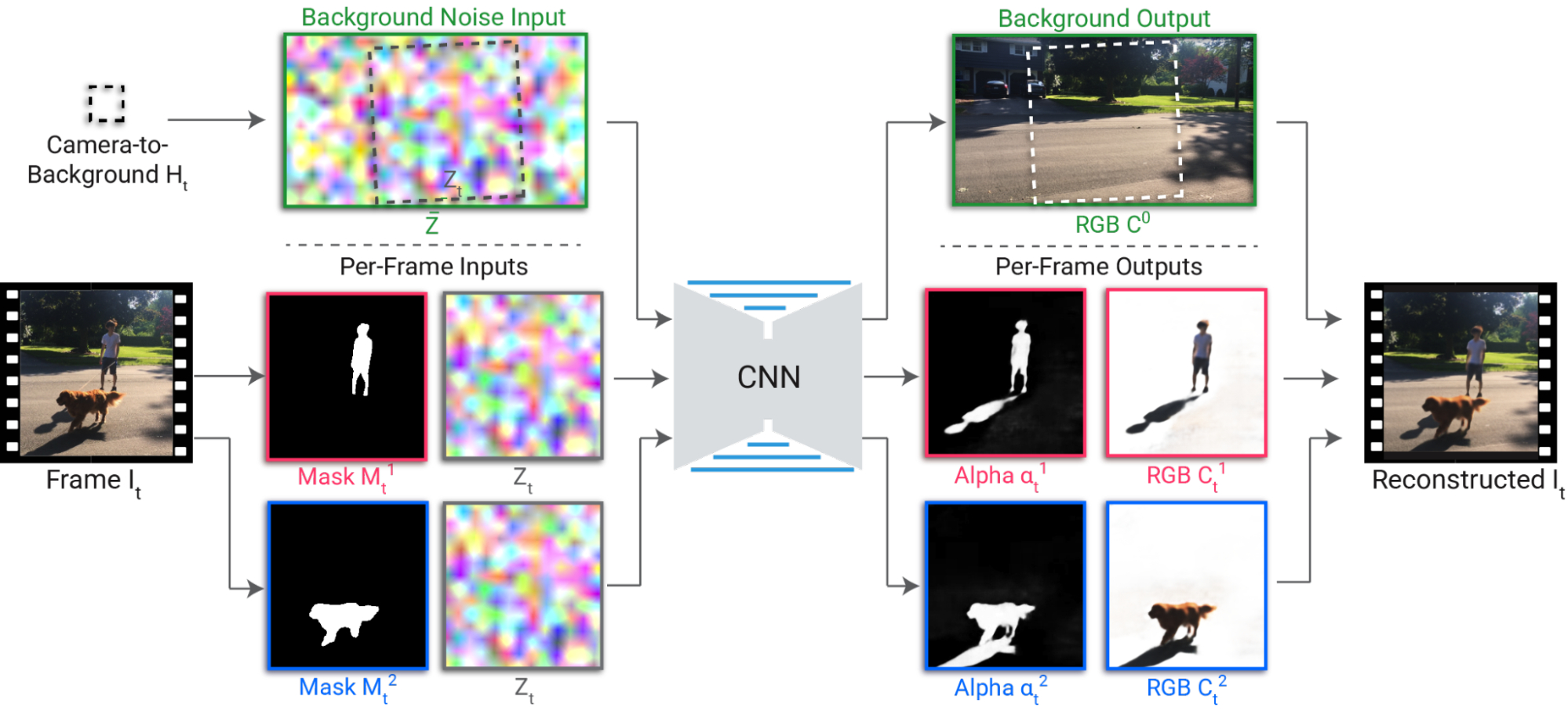
To train the Portrait Matting model, Google utilizes a custom volumetric capture system that captures detailed data about the subject's appearance from multiple perspectives. This comprehensive dataset provides the model with a rich source of information to learn from, enabling it to estimate high-resolution alpha mattes accurately.
Omnimattes: Unleashing Creativity in Video Editing
In video editing, Google has introduced an innovative approach called Omnimattes, which opens up a world of creative possibilities. Omnimattes allows users to capture and manipulate partially-transparent effects, such as reflections and splashes, in their videos, taking editing to new heights of creativity and visual appeal.
Capturing Partially-Transparent Effects


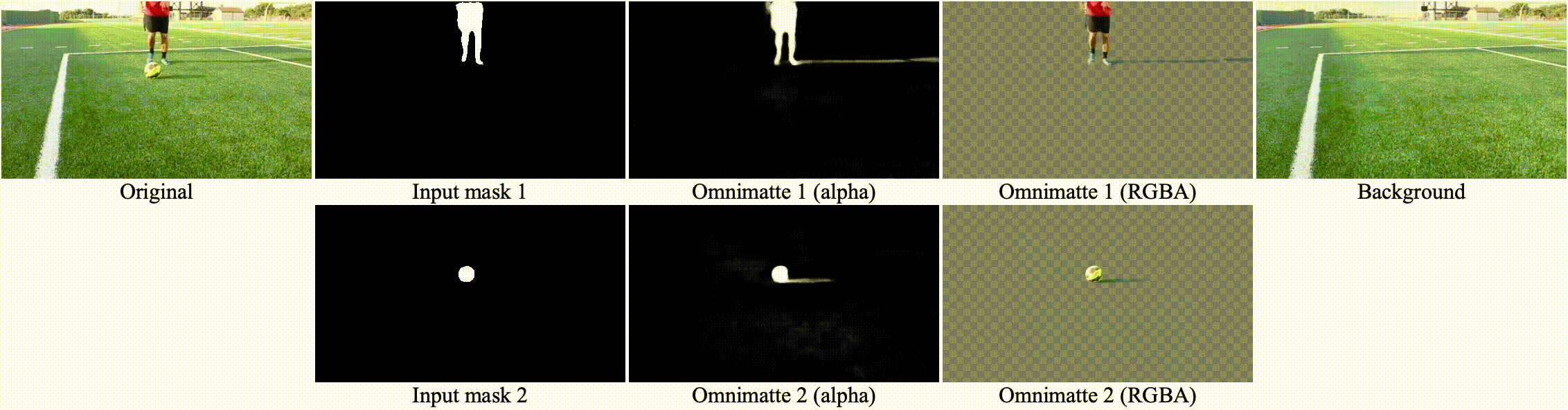
Omnimattes enables video creators to capture and emphasize the beauty of partially-transparent effects like reflections, splashes, and more. These effects add depth and visual interest to videos, making them more engaging and immersive. With Omnimattes, users can push the boundaries of video editing and unleash their creativity by incorporating these captivating elements into their videos.
The Power of Layers: Separating Videos
At the heart of Omnimattes lies the ability to separate videos into distinct layers. By decomposing a video into subject and background layers, users gain unprecedented control over the elements within their footage.
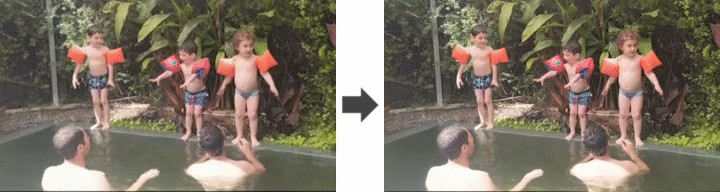
This separation allows for advanced editing techniques like object removal, duplication, and retiming, enhancing the overall quality and impact of the final video.
Unleashing the Power of HDR+
HDR+ is a remarkable feature of the Pixel Camera that empowers users to capture scenes with a wide brightness range, preserving detail even in challenging lighting conditions. This advanced technology utilizes multiple underexposed shots and intelligent mathematical calculations to create stunning images with balanced exposure and reduced noise.
Capturing Scenes with a Wide Brightness Range
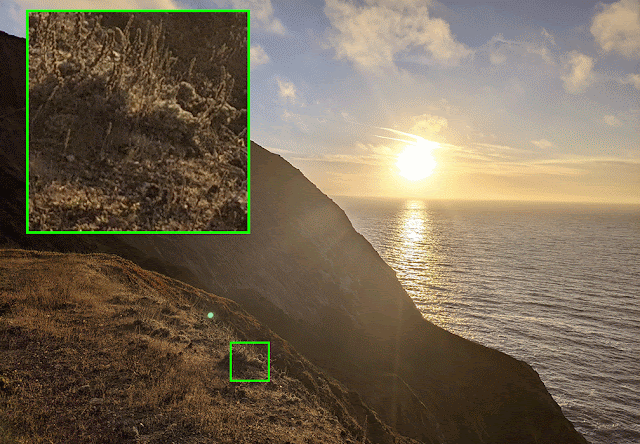
With HDR+ mode, the Pixel Camera can capture scenes that have a significant disparity in brightness levels. Whether it's a bright sky contrasting with a shadowy landscape or a well-lit subject against a backlit background, HDR+ intelligently combines multiple underexposed shots to bring out the details in both the highlights and shadows.
This results in photos that showcase a wide dynamic range, allowing every element of the scene to be captured with remarkable clarity and richness.
Live HDR+: Real-Time Preview
The Pixel 4 and 4a take HDR+ a step further with the introduction of Live HDR+. This feature provides users with a real-time preview of the final image with HDR+ applied.
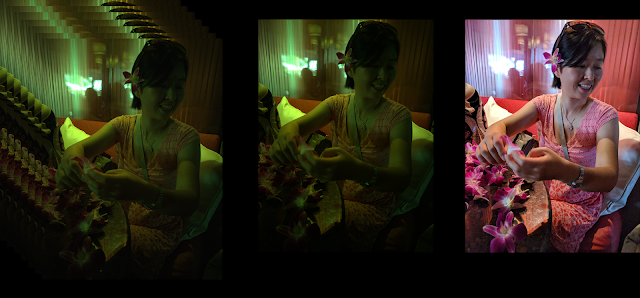
By seeing the enhanced result before pressing the shutter, users can make informed decisions and ensure that their photos turn out just the way they envision them. Live HDR+ gives users greater control over their shots, allowing them to fine-tune the composition and exposure settings for the best possible outcome.
Dual Exposure Controls: Shadow and Highlight Adjustments
To further enhance the photography experience, the Pixel Camera offers dual exposure controls that allow users to make separate adjustments for shadows and highlights. This level of control empowers photographers to fine-tune the image and achieve the perfect balance between the darker and brighter areas of the photo.
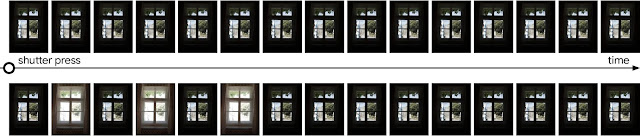
Whether it's preserving shadow detail or preventing highlight blowouts, the dual exposure controls provide a versatile toolset to ensure that every shot is precisely tailored to the photographer's vision.
Pushing the Boundaries with Super Res Zoom and Cinematic Photos
In addition to its impressive array of features, the Pixel camera also introduces the concept of Cinematic Photos and Super Res, Zoomallowing users to bring their still images to life and create visually captivating scenes.
Simulating Camera Motion and Parallax
Cinematic Photos offer a unique way to simulate camera motion and parallax within a single still image. By adding subtle movements and shifts in perspective, these photos become more dynamic and engaging, evoking a sense of motion that goes beyond the static nature of traditional photographs.
This innovative feature truly unlocks a new level of creativity, enabling users to transform their still images into immersive visual experiences.
Depth Estimation and Monocular Cues
To achieve the cinematic effect, the Pixel camera utilizes advanced techniques such as depth estimation and monocular cues. Depth estimation allows the camera to understand the spatial relationships within the scene, identifying different layers of depth and enabling the creation of realistic depth-of-field effects.
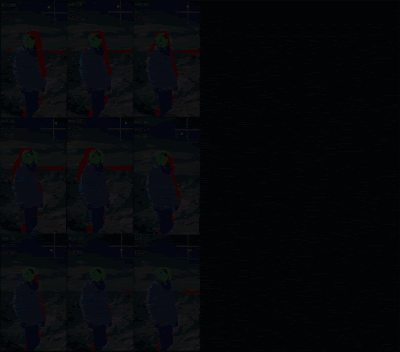
This adds a sense of depth and dimensionality to the image, making it visually compelling and enhancing the overall cinematic experience.
In addition to depth estimation, monocular cues play a crucial role in creating cinematic photos. Monocular cues refer to visual cues that our brain uses to perceive depth, even with just one eye.
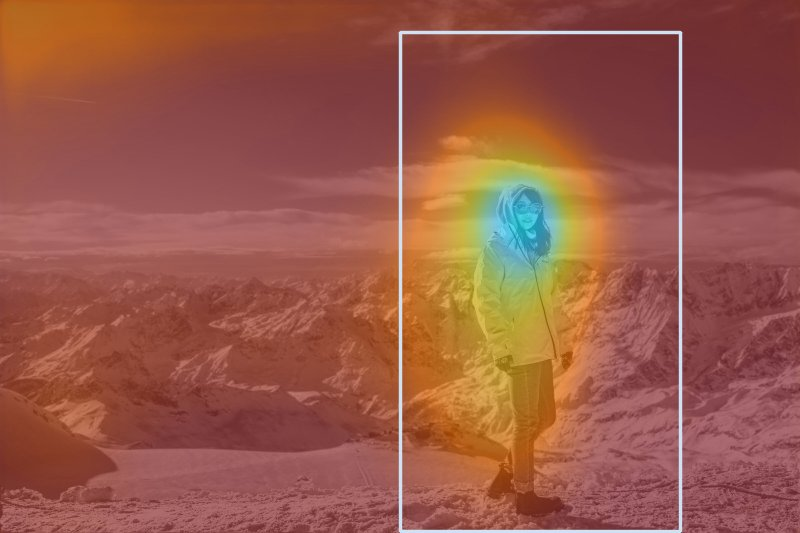
By leveraging these cues, the Pixel camera can intelligently apply depth effects and simulate the parallax effect, making the subject of the photo stand out and giving it a sense of three-dimensionality.
Merging Frames for Improved Resolution
Super Res Zoom works by merging multiple frames taken in quick succession when zooming in on a subject. By analyzing these frames and intelligently aligning them, the camera is able to create a final image with enhanced resolution and clarity.
This technique effectively combines the information from different frames to create a single high-resolution image, surpassing the limitations of traditional zoom methods.
Leveraging Open Images and DeepLab-v3+
To further advance the capabilities of the Pixel camera, Google harnesses the power of open-source resources such as Open Images and DeepLab-v3+. These tools are crucial in improving semantic image segmentation and enhancing the overall photography experience.
Open Images: An Annotated Image Collection
Open Image is a remarkable collection of annotated images that is a valuable resource for training machine learning models. It provides images and detailed labels, and segmentation masks that identify and outline various objects and elements within the images. This comprehensive dataset enables the Pixel camera to understand better and analyze images' content, allowing for more accurate and precise segmentation.
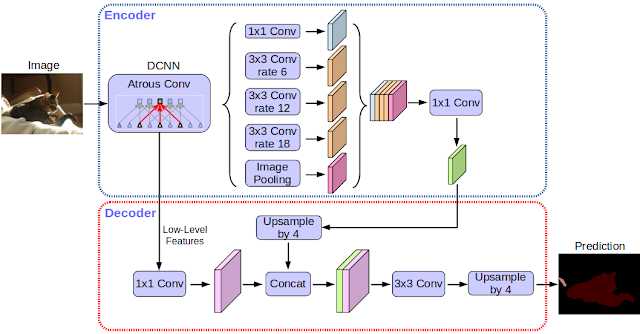
Using Open Images, Google can build a robust dataset encompassing a wide range of scenes, objects, and scenarios. This dataset becomes an invaluable asset in training the algorithms and models that power the Pixel camera, helping it to excel in image recognition and object detection and, ultimately, delivering stunning results.
DeepLab-v3+: Advancing Semantic Image Segmentation
DeepLab-v3+ is an advanced semantic image segmentation model implemented in TensorFlow, a popular machine learning framework. This model plays a pivotal role in refining the Pixel camera's image segmentation capabilities, enabling it to distinguish and delineate different elements within an image accurately.
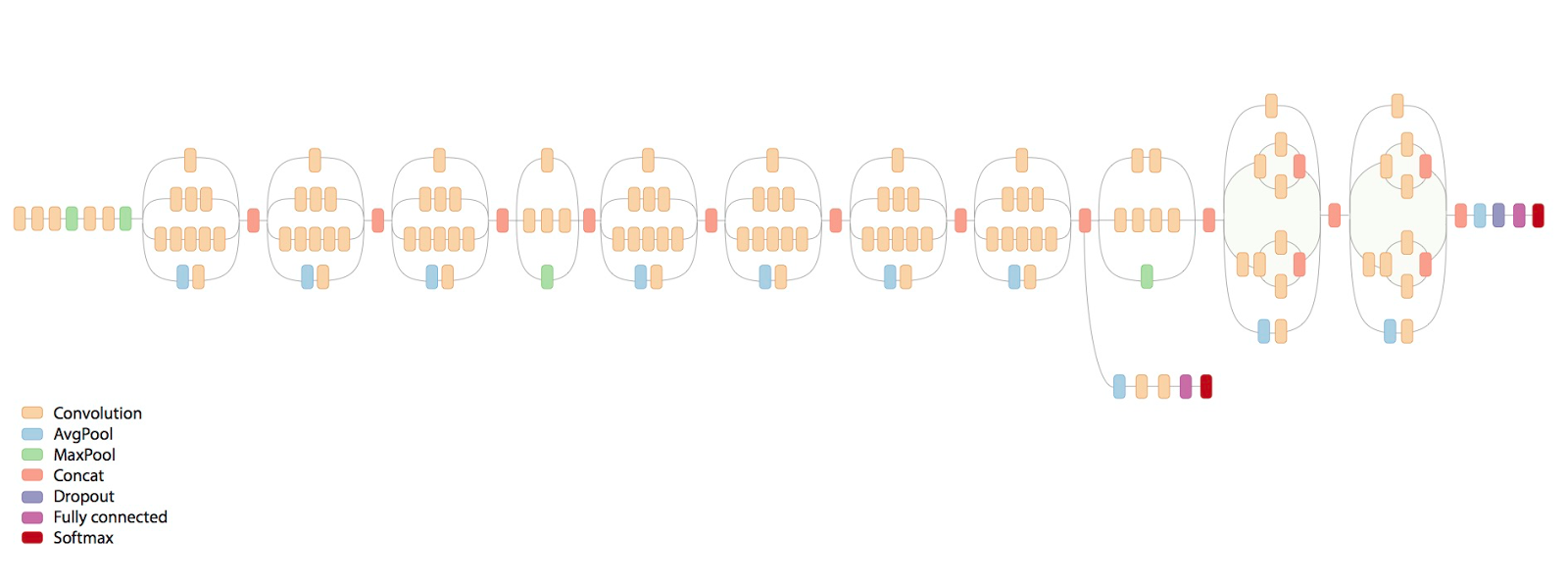
DeepLab-v3+ leverages the power of deep learning, utilizing neural networks to process and analyze images at a pixel-level granularity. The model can capture intricate details and effectively segment objects within an image by incorporating sophisticated techniques like dilated convolutions and atrous spatial pyramid pooling.
The Pixel camera redefines smartphone photography, showcasing the power of software-driven innovation. It empowers users to capture stunning images, push their creative boundaries, and immortalize their most cherished moments.
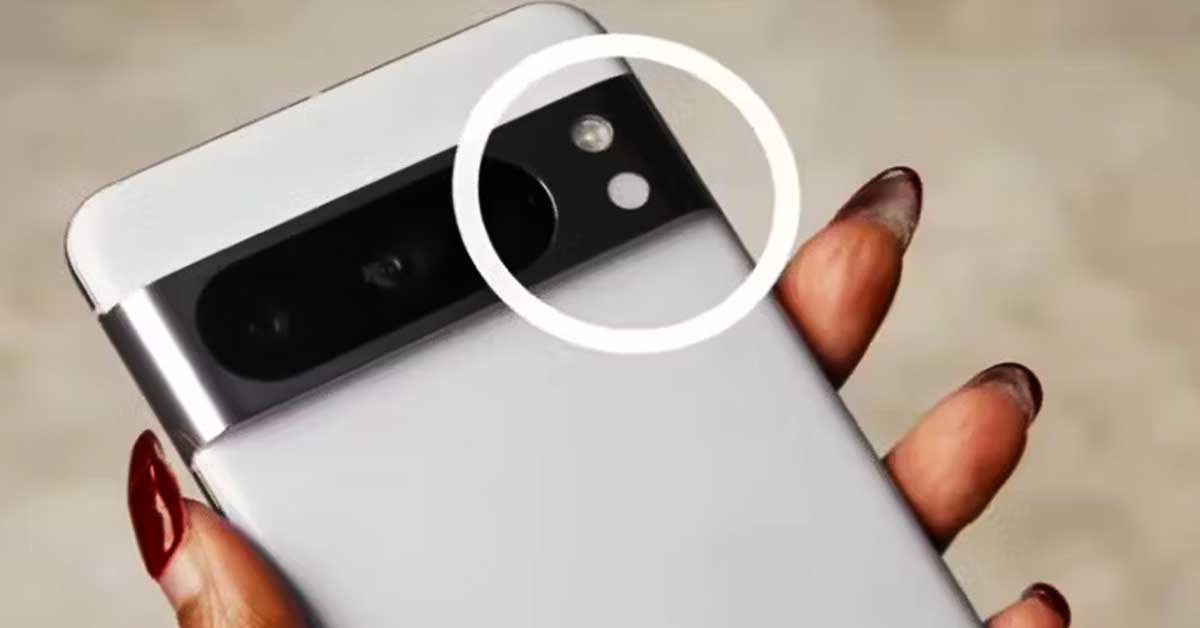
With each advancement, Google sets new standards for excellence in computational photography, inspiring us to see the world through a technologically advanced and artistically compelling lens.
Sources: googleblog.com / techniknews.net / arxiv.org /acm.org / blog.x.company / cambridgeincolour.com