The rapid advancement of generative AI technology, exemplified by OpenAI's ChatGPT, has sparked a revolution in various industries. With as many as half the employees in leading global companies already utilizing generative AI in their workflows and a surge of new products incorporating this technology, the age of generative AI has unquestionably arrived.
However, a pressing question arises as AI-generated content becomes increasingly prevalent on the internet: What are the consequences when AI models are trained on AI-generated data instead of primarily human-generated content?
In a recent study published in the open-access journal arXiv, researchers from the UK and Canada shed light on this issue. Their findings suggest that using model-generated content in training can lead to irreversible defects in the resulting models.
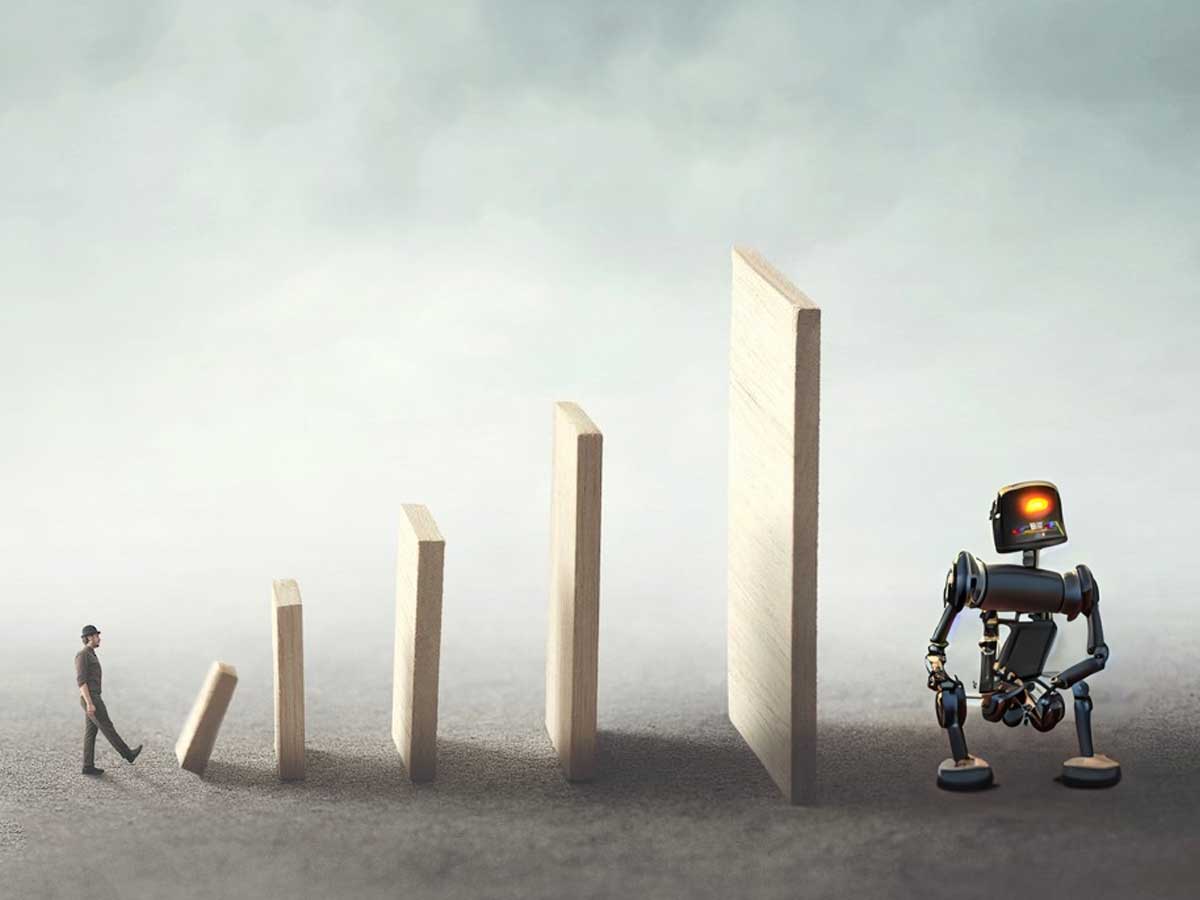
The researchers discovered that models exposed to AI-generated data over time suffer from a degenerative process known as "model collapse," causing them to forget the true underlying data distribution. The implications of this phenomenon are concerning for the future of generative AI technology.
The researchers focused on analyzing probability distributions for text-to-text and image-to-image AI generative models. They concluded that model collapse is an inevitable outcome when models learn from data produced by other models, even under nearly ideal conditions for long-term learning.
Model collapse manifests as a progressive deterioration in the model's performance, resulting in increased errors and a reduced variety of non-erroneous responses.
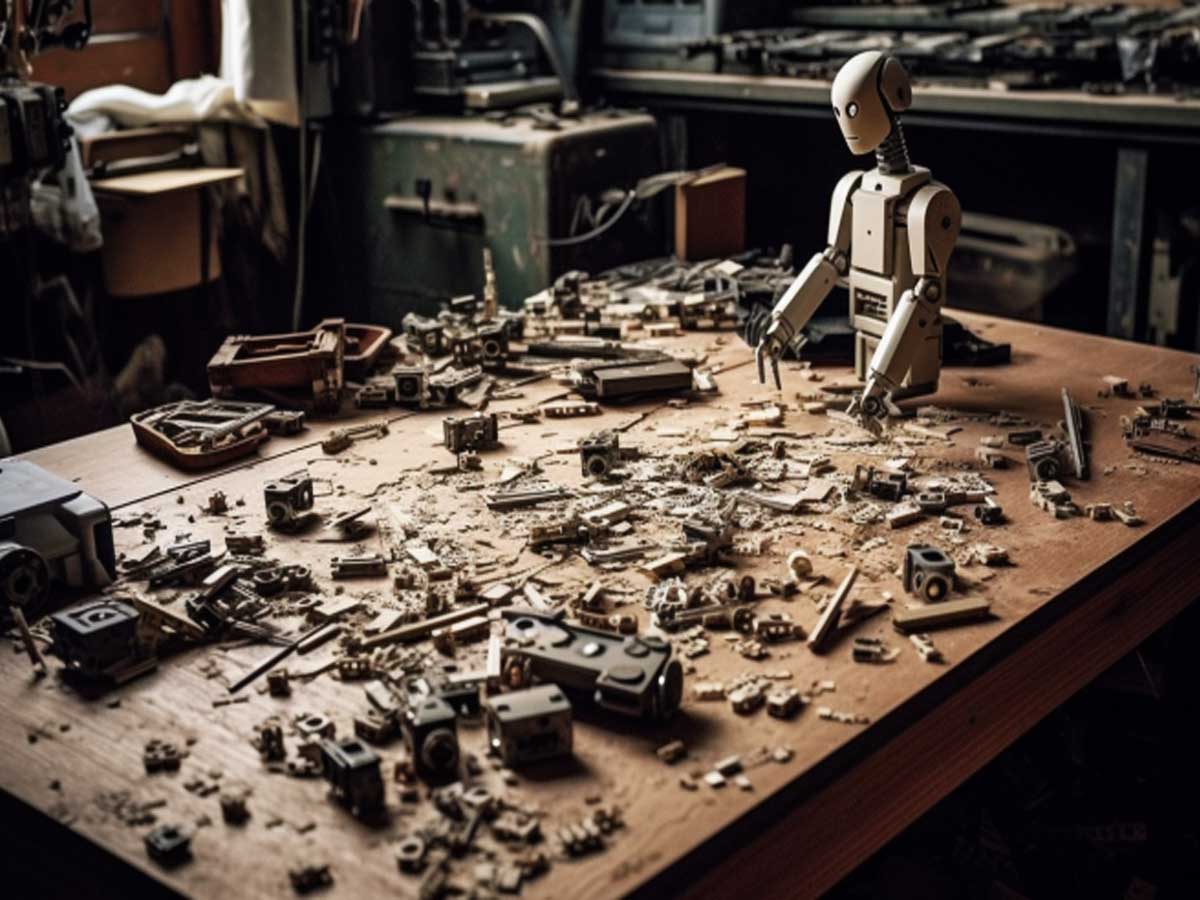
The speed at which model collapse occurs is astonishing. Models quickly need to remember a significant portion of the original data from which they initially learned. Consequently, the quality of the responses and content they generate diminishes, and they need more diversity in their output. This phenomenon raises concerns about the reliability and accuracy of AI-generated content as the prevalence of AI training on AI-generated data grows.
The contamination of training sets with AI-generated data is a key factor contributing to the model collapse. While original data created by humans represent the world fairly, including improbable occurrences, generative models tend to overfit popular data and misinterpret less common data.
To illustrate this problem, imagine training a machine learning model on a dataset of 100 cat pictures, with 10 cats with blue fur and 90 with yellow fur. The model may mistakenly represent blue cats as more yellowish than they are, resulting in the generation of green-cat images.
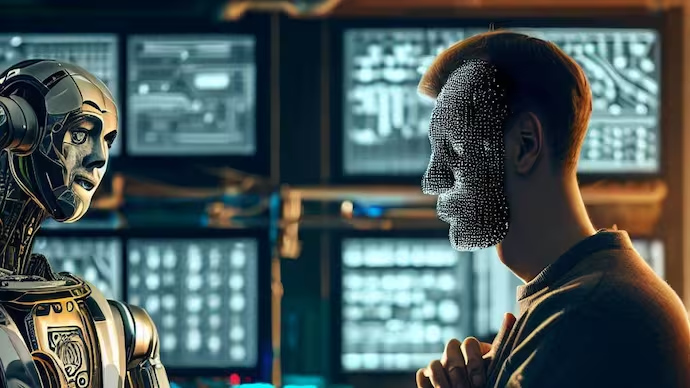
Over successive training cycles, the model's representation of blue fur erodes, eventually turning the blue cats greenish and, ultimately, yellow. This progressive distortion and loss of minority data characteristics define model collapse.
To mitigate this issue, it is crucial to ensure fair representation of minority groups in datasets, both in quantity and accurate portrayal of distinctive features. However, achieving this goal is challenging due to the models' difficulty learning from rare events.
Model collapse distorts the perception of reality within AI models and carries serious implications in various contexts. Even when researchers attempted to restrict models from generating excessive repetitions, they found that model collapse still occurred. The models generated erroneous responses in these cases to avoid repeating data too frequently.
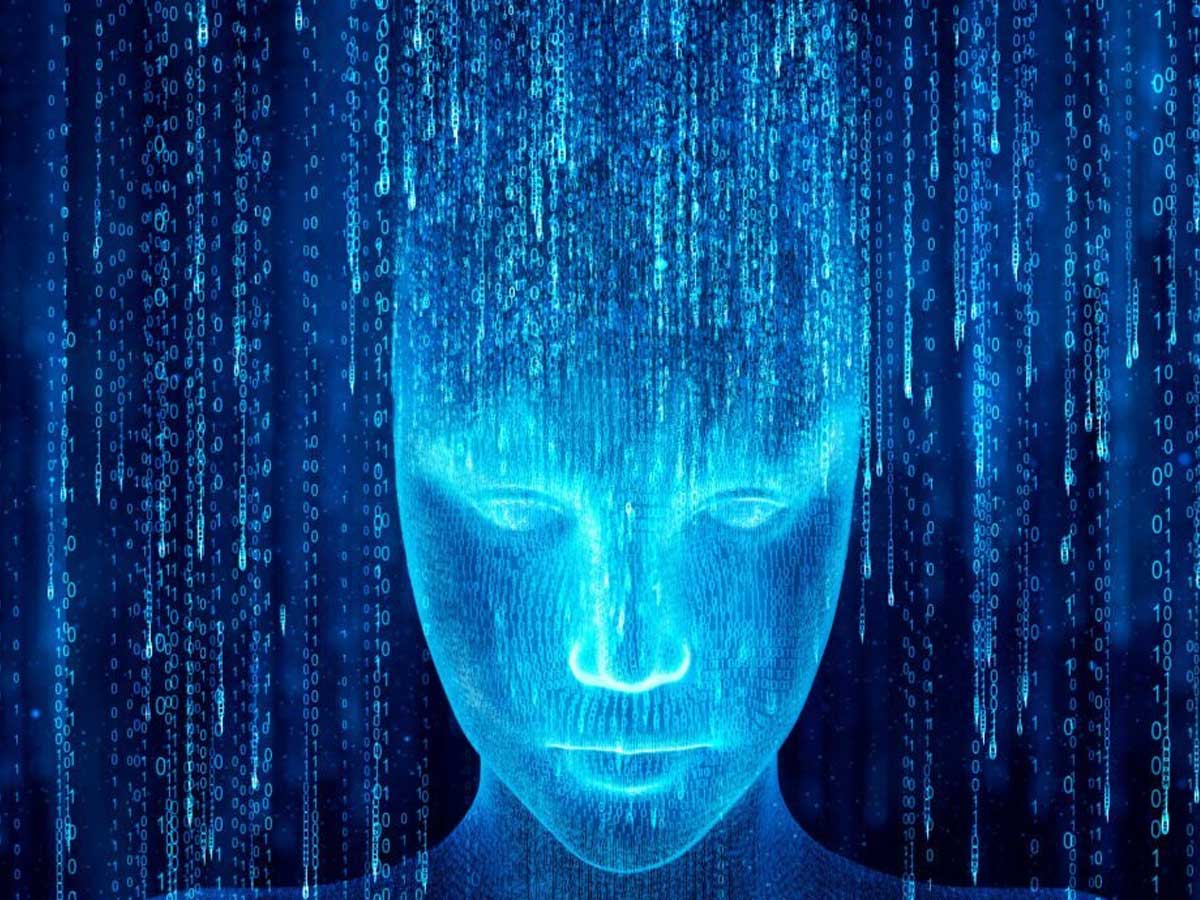
Moreover, the researchers highlight potential discrimination based on gender, ethnicity, or other sensitive attributes. If generative AI models learn to produce responses biased toward one race while "forgetting" the existence of others, it could perpetuate unfair biases and exacerbate societal inequalities.
It is important to distinguish model collapse from "catastrophic forgetting," where models lose previously learned information. Model collapse involves models misinterpreting reality based on their reinforced beliefs rather than simply forgetting information. Even if
Subsequent generations of models are trained using only 10% of the original human-authored data, and model collapse still occurs, albeit at a slower pace.
Thankfully, strategies are available to mitigate model collapse, even with existing transformers and large language models (LLMs). The researchers propose two specific approaches.
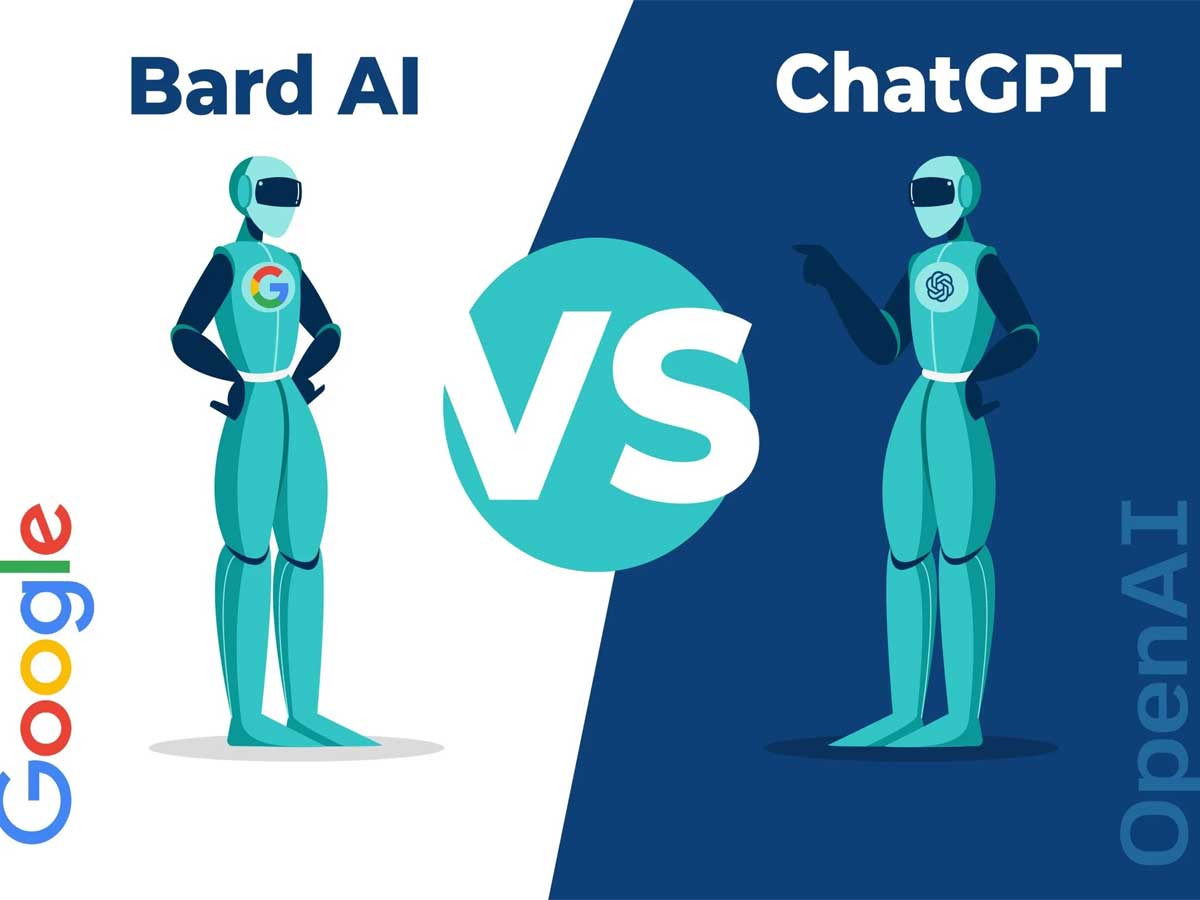
The first involves preserving a prestigious copy of the exclusively or predominantly human-produced original dataset, ensuring it remains uncontaminated by AI-generated data. Periodic retraining or a complete model refresh using this pristine dataset can help prevent model collapse.
The second approach to combat degradation in response quality and reduce errors and repetitions from AI models is introducing new, clean, human-generated datasets during training.
However, implementing this solution requires a reliable and large-scale mechanism to differentiate between AI-generated and human-generated content. Currently, such effort is only somewhat present on the internet.
To address model collapse effectively, minority groups from the original data must be fairly represented in subsequent datasets. Achieving this is a non-trivial task that requires meticulous backup and coverage of all possible corner cases.
When evaluating model performance, utilizing the data that the model is expected to work on, including the most unlikely data cases, is essential. It is important to emphasize that representing improbable data appropriately does not mean oversampling it but rather ensuring its fair inclusion.
Although including old and new data increases the cost of training, it helps counteract model collapse to some degree.
While model collapse poses challenges for generative AI technology and companies aiming to capitalize on it, there is a silver lining for human content creators. The researchers conclude that in a future inundated with generative AI tools and their content, human-created content will become even more valuable than it is today.
Human-generated content holds intrinsic value as a source of new training data for AI models. As generative AI continues to evolve, ensuring the integrity and reliability of these models over time will be crucial for their continued improvement and success.
The rise of generative AI technology brings both promise and challenges. The problem of model collapse poses a significant hurdle to overcome. The irreversible defects that emerge when models are trained on AI-generated data compromise their performance and distort their perception of reality.
However, strategies are available to combat model collapse, including preserving pristine human-authored datasets and introducing clean human-generated data during training. As the AI industry and users move forward, it is crucial to address the issue of model collapse to ensure the continued advancement and responsible utilization of generative AI technology.
Sources: venturebeat.com / arxiv.org