We live in an AI era where everything is changing super fast, and without knowing everything, every file is connected to AI o. r artificial intelligence. Back in 2016, Gartner Research got creative with language and coined "AIOps." They took "Algorithmic IT Operations" and gave us this quicker, more digestible version.
It wasn't just a new term; they were reimagining IT Operations Analytics (ITOA).
A year later, Gartner did a little twist on the phrase, turning it into "Artificial Intelligence for IT Operations." This is where everything started. So, let's talk about AIOps and how it will affect you.
What is AIOps
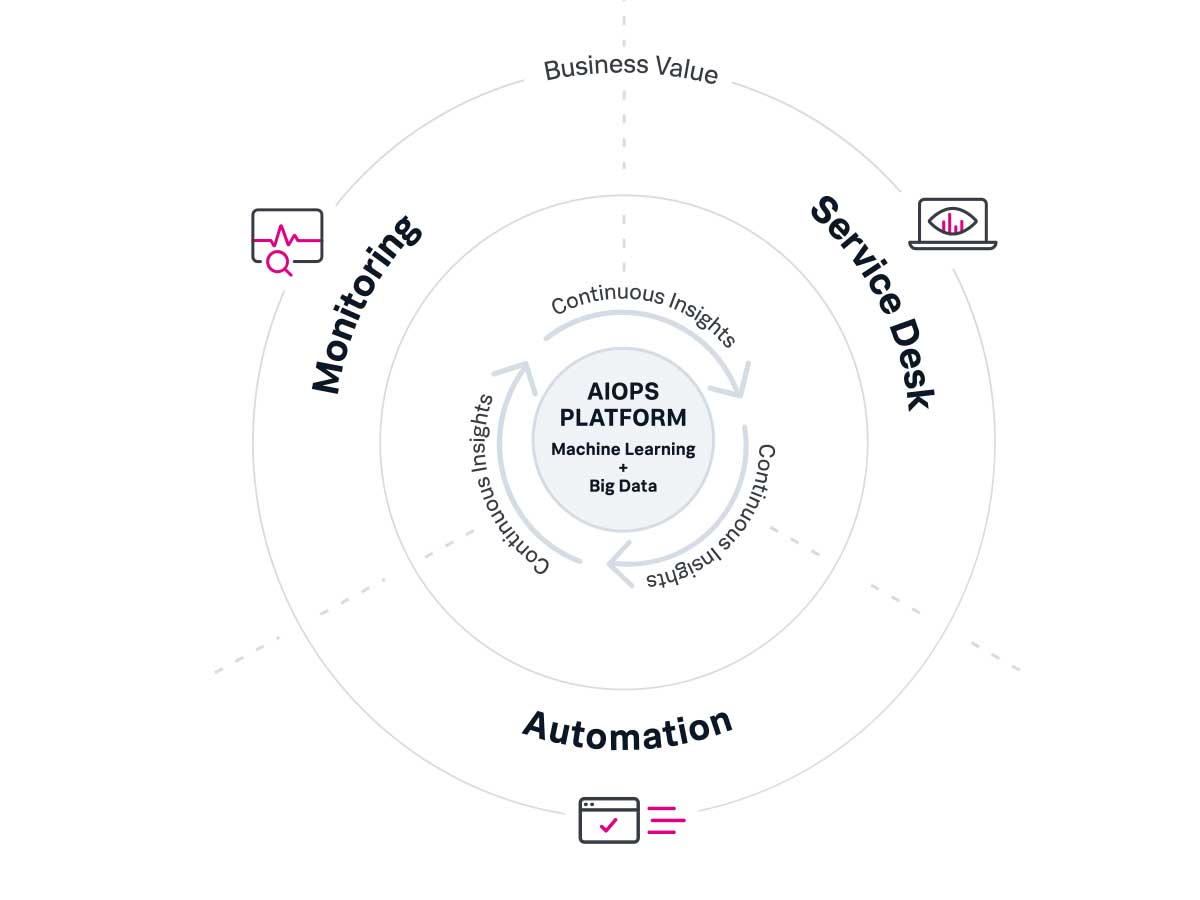
AIOps, or Artificial Intelligence for IT Operations, refers to the comprehensive application of AI technologies like big data analytics and machine learning to identify, detect, and address prevalent issues in IT automatically.
In large-scale enterprises, particularly with modern distributed architectures like containers, microservices, and multi-cloud environments, there's a vast quantity of log and performance data generated.
This data volume can overwhelm IT teams, making pinpointing and rectifying incidents challenging. AIOps leverage this extensive data to oversee assets and understand the interconnections within and external to IT infrastructures.
An AIOps platform should provide enterprises with the ability to do the following:
- Automating Routine Practices: AIOps step in to handle user requests and noncritical IT system alerts. Help desk systems benefit hugely here, as they can now fulfill user requests for resource provisioning without human intervention.
- Enhanced Issue Recognition and Prioritization: Ability to quickly and accurately recognize serious issues. This is crucial in scenarios where IT professionals might overlook subtle yet critical events, such as an unusual download or a process starting on a critical server.
AIOps prioritizes these events, treating them as potential attacks or infections while intelligently deprioritizing less critical issues like known malware events on non-essential systems.
- Streamlining Data Center Operations: AIOps significantly streamline interactions between data center groups and teams.
It eliminates much of the manual data sharing and processing, providing functional IT groups with the exact data and insights they need.
This is achieved through AI-enabled operations, including monitoring and automation, which ensures that relevant analysis and monitoring data are distributed efficiently from a large pool of resource metrics.
Basically, AIOps takes all those different manual tools you've got scattered around and pulls them into one intelligent, automated platform. This means IT operations teams can respond to issues way faster, and sometimes even before they happen. It's about giving them that full picture, that end-to-end visibility and context they need to stay ahead.
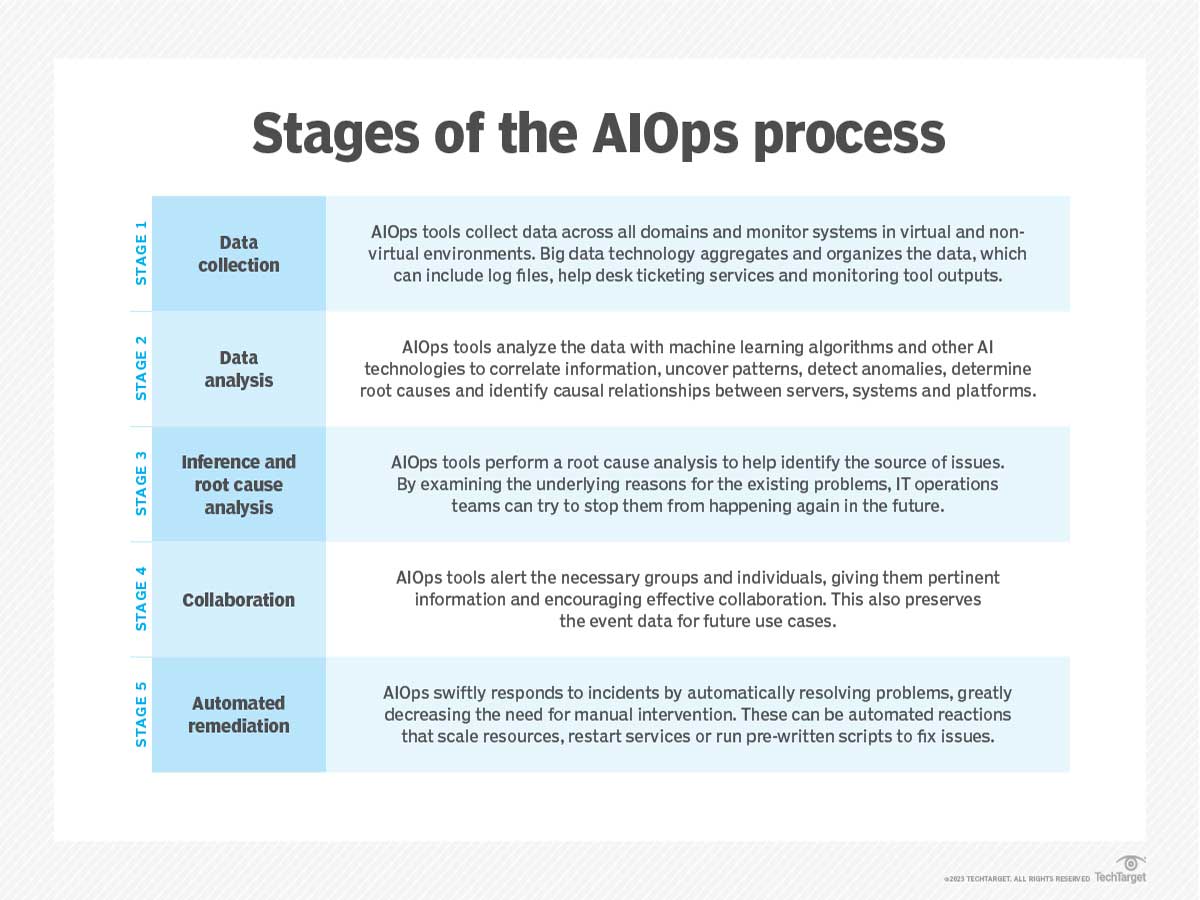
AIOps also stepped in to smooth out the rough spots between a really complex IT landscape and teams that used to work in their own little bubbles.
This is super important because users want to avoid any hiccups in their app performance or availability. They expect things to just work.
How does actually AIOps Functions
To explain that better, let's break it down into several sections. With that, you'll be able to understand this mechanism better.
Data Collection: AIOps platforms gather diverse data types, including application logs, event and configuration data, incidents, performance metrics, and network traffic.
This encompasses both structured data (like databases) and unstructured data (such as social media posts and documents).
Data Analysis: Utilizing machine learning algorithms, such as anomaly and pattern detection, along with predictive analytics, AIOps examines the collected data.
It distinguishes genuine issues from mere noise or false alarms, ensuring that IT staff focus on real problems.
Inference and Root Cause Analysis: AIOps conducts thorough root cause analysis, aiding in pinpointing the origins of issues. Understanding the root causes enables IT operations teams to work on preventing similar problems in the future.
Collaboration: After root cause analysis, AIOps alerts relevant teams and individuals, equipping them with necessary information. This fosters efficient collaboration, even over geographical distances, and helps preserve event data for future reference in similar situations.
Automated Remediation: AIOps can resolve issues autonomously, minimizing the need for manual intervention and accelerating response times. Automated actions might include resource scaling, service restarts, or executing specific scripts to rectify issues.
Adopting AIOps
The path to adopting or Implementing AIOps varies from one organization to another. After evaluating your current position on the AIOps journey, you can begin combining tools that assist teams in monitoring, forecasting, and promptly addressing IT operational challenges.
When evaluating tools to enhance AIOps within your organization, it's essential to verify that they encompass the following features:
Observability
When it comes to Observability, it involves choosing top-notch software tools that are experts in digesting, combining, and analyzing a whole lot of performance data from your applications and hardware. These tools are vital for keeping tabs on things, helping you troubleshoot, and making sure everything's beating along nicely.
They play a big part in meeting customer experience expectations and sticking to those SLAs. While they're not the fix-it type, they do a stellar job at alerting your team about potential issues by gathering and processing IT data from various places.
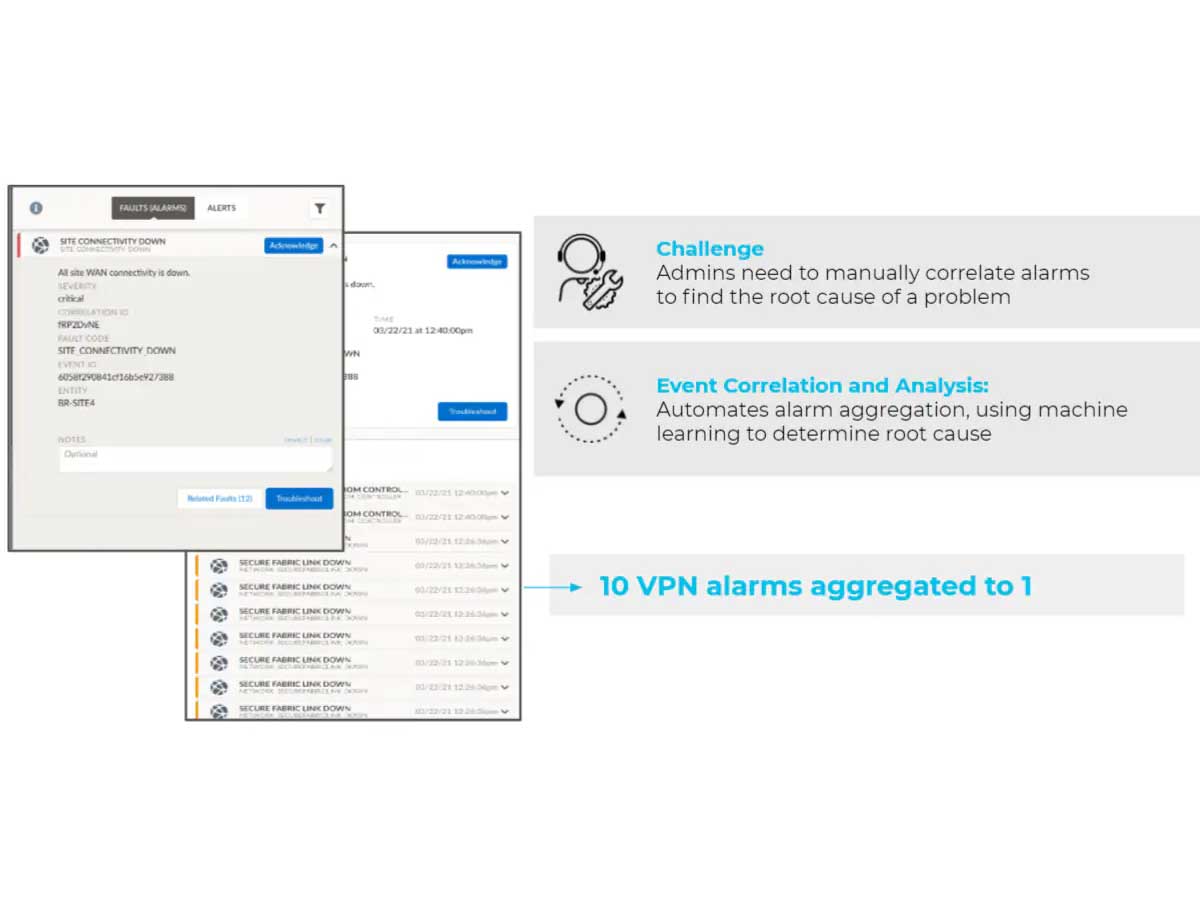
Predictive Analytics
Predictive Analytics in AIOps is the brainy part of the operation. These solutions dive into the data, analyzing and connecting the dots for sharper insights and even some automated decision-making. This helps you keep everything under control and ensures your applications are running smoothly.
This feature helps reduce the time it takes to spot problems, cut down on downtime, and keep those annoying incidents and tickets to a minimum. Plus, it's got the interlligence for automatic anomaly detection, alerting, and offering solutions, which is a huge win.
Proactive Response
The proactive Response aspect of AIOps is where things get proactive. These solutions don't just wait around for problems to happen; they're looking for potential issues like slowdowns and outages and dealing with them in real time.
By analyzing application performance metrics, they can spot patterns and trends that might spell trouble and jump into action before things go south. This means they can kick off the right processes to sort out issues. It's all about intelligent automation, improving your mean time to detection (MTTD), and giving your IT operations a smart, automatic helping hand.
AIOps use cases
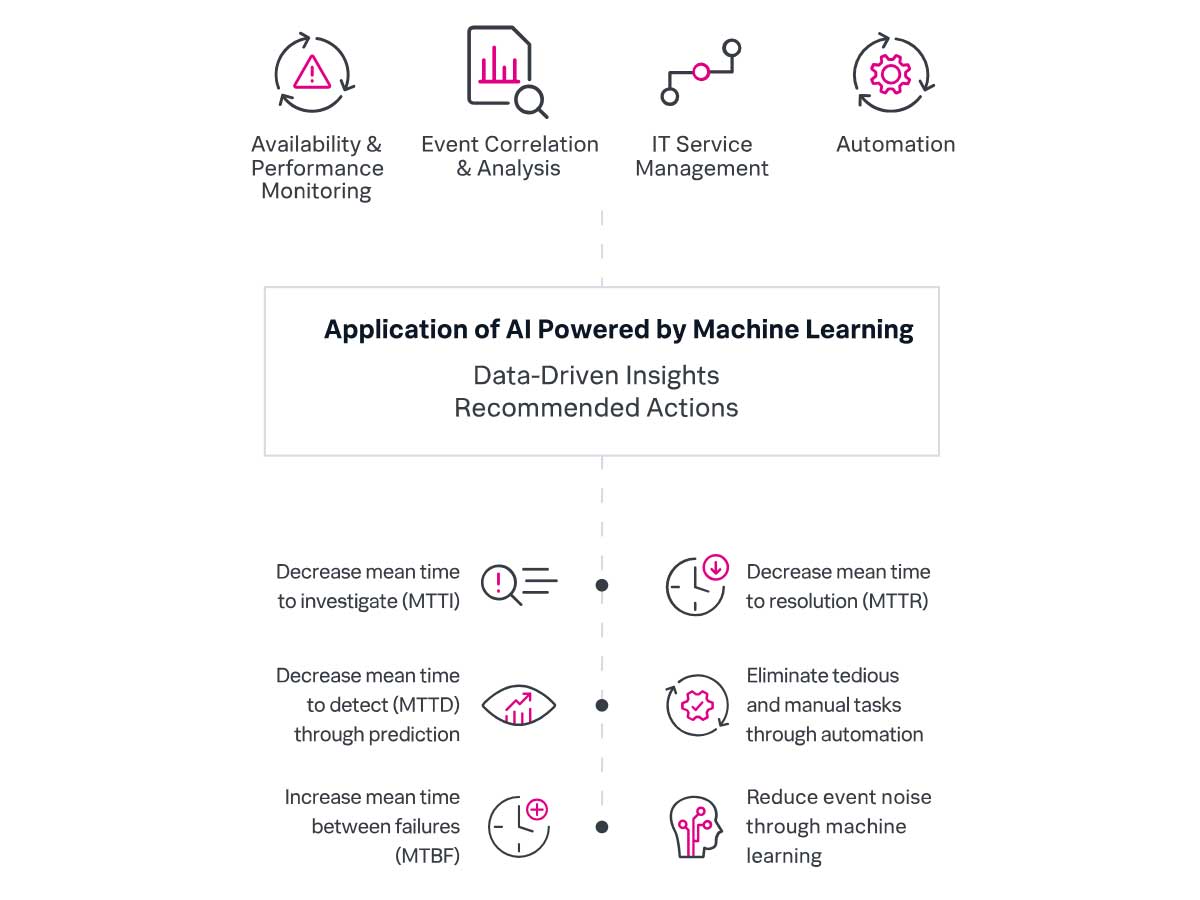
AIOps is often adopted by organizations that leverage DevOps or cloud computing, as well as in large enterprises with intricate systems.
It provides DevOps teams with deeper insights into their IT environments and the high volume of data, enhancing the operations teams' understanding of production changes.
Here are some very common use cases of AIOps
- Mitigating Risks in Hybrid Cloud Environments: Hybrid cloud structures, known for their intricate architectures and component interactions, can pose efficiency and accuracy risks. AIOps addresses these by overcoming operational limitations in hybrid cloud settings.
- Process Automation: In substantial companies with complex IT landscapes, AIOps are critical in automating processes, early problem detection, and facilitating smoother communication between teams.
- Anomaly Detection: Leveraging AI, AIOps efficiently scans vast historical data sets, rapidly categorizing patterns, and surpassing human capabilities in identifying issues and their root causes.
- Performance Monitoring: In modern applications, identifying supporting resources can be challenging due to multiple abstraction layers. AIOps is a storage, virtualization, and cloud infrastructure monitoring tool that tracks consumption, availability, and response time metrics. It also excels in event correlation, enhancing access to aggregated information.
- Understanding Customer Needs: By collecting real-time data from customer interactions, AIOps aids businesses in comprehending client demands, leading to an enhanced customer experience. This data can also guide product adjustments based on customer feedback, elevating satisfaction levels.
- Threat Detection: AIOps aid in spotting security risks, unusual activities, and signs of malicious behavior. It analyzes log data, network traffic, and security events in real-time, enabling swift incident responses and reducing threats and intrusions.
- Implementing DevOps: While DevOps accelerates development by empowering development teams to provision and modify infrastructure, IT management of this infrastructure remains essential.
AIOps enhances this process by offering the necessary visibility and automation, enabling IT to back DevOps effectively without significant extra management workload.
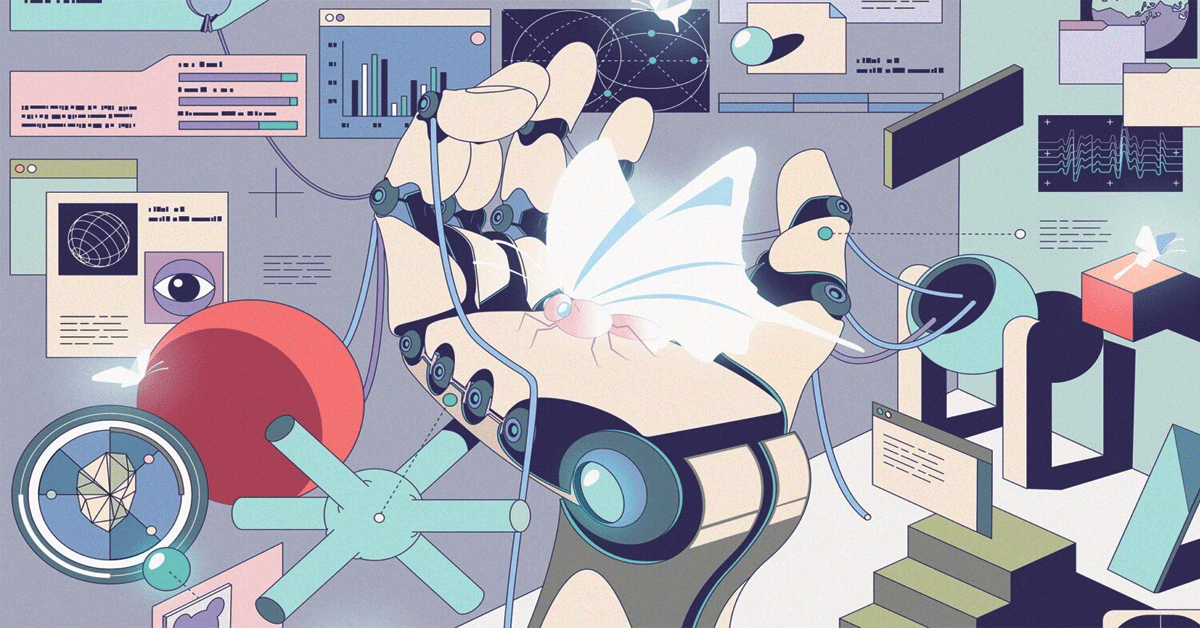
AIOps can provide significant business benefits to an organization.
Healthcare IT (HIT):
- Ensuring the security of electronic personal healthcare information (ePHI) in line with the Health Insurance Portability and Accountability Act (HIPAA).
- Mitigating risks associated with mobile networking and bring-your-own-device (BYOD) policies among healthcare professionals.
- Guarding against ransomware attacks, which often target healthcare entities.
- Facilitating the availability of extensive data, both internal and external, for research and diagnostic purposes.
Manufacturing IT:
- AIOps streamlines the gathering and analysis of diverse data stemming from the integration of supply chain, plant operations, and product and service lifecycle management.
- It enables real-time monitoring of factory machinery, consolidating data like manufacturing cycle times, machine-specific quality yields, capacity utilization, and supplier quality levels.
- It aids in averting production delays and conducts troubleshooting using historical data and AI-driven predictive analytics, thereby safeguarding revenue and enhancing customer satisfaction.
- AIOps also contribute to predictive maintenance, addressing machine issues preemptively.
- It optimizes data use for more efficient supply chain management systems.
IT for Financial Services:
- Thwarting increasingly complex security breaches and cybercrime.
- Utilizing customer data to fuel marketing strategies and growth opportunities.
- Analyzing historical customer data for more precise revenue growth forecasting.
- Maintaining data security and adhering to regulatory compliance.
- Establishing a framework for consolidating large, varied data sets to support emerging technologies like blockchain.
- Keeping pace with consumer expectations for mobile and digital banking experiences.
- Enhancing network speed and performance.
What are AIOps technologies?
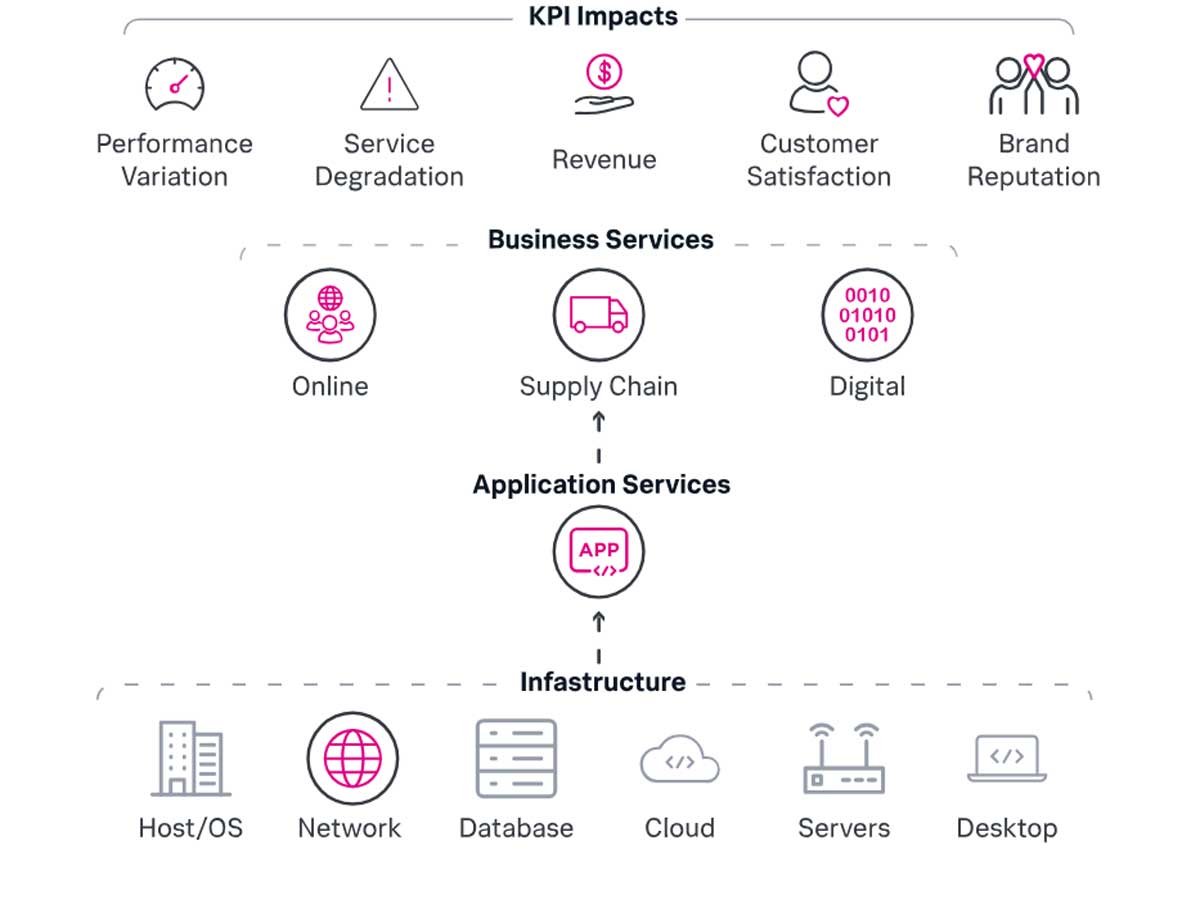
AIOps combines a variety of AI approaches, such as data processing, aggregation, sophisticated analytics, algorithms, automation and orchestration, machine learning, and visualization. These technologies are generally, we can say, reasonably well-established and mature.
Machine Learning in AIOps: ML employs algorithms that allow computer systems to learn from extensive data sets and adjust to new data. It encompasses various methods like supervised and unsupervised learning, reinforcement learning, and deep learning.
Within AIOps, ML is commonly utilized for tasks like anomaly detection, root cause analysis, event correlation, and predictive analytics.
Analytics within AIOps: AIOps gathers data from sources like log files, metrics, monitoring tools, and help desk ticketing systems. Analytical techniques interpret this raw data to generate new information and metadata.
This process helps in reducing irrelevant data and identifying trends and patterns. These insights are crucial for pinpointing and isolating issues, forecasting capacity needs, and managing various events.
Role of Algorithms in AIOps: Algorithms are vital in AIOps for embedding the organization's IT expertise, business policies, and objectives. They guide an AIOps platform to produce preferred actions or results, such as prioritizing security events or optimizing application performance.
These algorithms lay the groundwork for machine learning, helping the platform establish a norm for behaviors and activities and adapt to environmental data changes over time.
Automation in AIOps: Automation is fundamental in enabling AIOps tools to act. Automated processes are triggered by analytics and machine learning findings.
For instance, if predictive analytics and ML indicate that an application requires additional storage, the system automatically initiates a process to add storage following algorithm-based rules.
Visualization in AIOps: Visualization tools present user-friendly dashboards, reports, graphics, and other formats, allowing users to monitor changes and events.
These visual tools enable human users to make decisions based on the information, which might be beyond the decision-making scope of AIOps software.
Advantages and Disadvantages of AIOps
Like any other technology or thing, there are some benefits and advantages to this technology, but as always, there are some drawbacks and disadvantages as well. Let's talk about that.
Benefits & Advantages of AIOps
Efficiency in Time Management with AIOps: A key benefit is the drastic reduction in time IT staff spend on routine alerts. AIOps platforms evolve through machine learning, utilizing algorithms and accumulated knowledge to enhance software behavior.
Additionally, AIOps notably cuts down the mean time to resolution (MTTR). By analyzing IT operations noise and correlating data, AIOps surpasses human capabilities in speed and accuracy.
Ongoing Automated Monitoring and Cost Reduction: AIOps tools offer continuous, 24/7 monitoring, allowing human IT staff to focus on more complex tasks.
This not only boosts business performance and stability but also reduces operational costs significantly. Automated identification of operational issues and pre-programmed response scripts lead to substantial savings and strategic resource allocation.
Facilitating Digital Transformation and Proactive Management: AIOps reduce IT incidents and repair time, supporting a more agile and secure digital infrastructure.
It plays a crucial role in transitioning to proactive and predictive management. Incorporating predictive analytics, AIOps enables IT teams to anticipate and address issues before they escalate.
Enhanced Visibility and Improved Collaboration: With AIOps, IT teams gain better visibility into infrastructure and applications, leading to proactive issue identification.
AIOps monitoring tools, through integrations, also enhance collaboration among DevOps, ITOps, governance, and security teams. This improved visibility and communication aid in faster decision-making and issue resolution.
Data Correlation and Analysis with AIOps: AIOps software excels in identifying causal links across systems and resources, clustering, and correlating diverse data sources for comprehensive analysis. This capability is crucial for in-depth root cause analysis and quick resolution of complex issues.
Enhancing Team Collaboration with AIOps: AIOps improves collaboration and workflows, providing customized reports and dashboards for better team understanding and interaction. The integration of AIOps into team dynamics elevates the overall employee experience by freeing staff for more innovative tasks.
Drawbacks & Disadvantages of AIOps
Data Accuracy and Quality: The effectiveness of AIOps heavily depends on the quality of data it processes and the sophistication of its algorithms. As AIOps is still emerging in terms of practical technology integration, it's crucial for organizations to maintain up-to-date and precise data.
Complexities in Deployment and Integration: Setting up, managing, and maintaining an AIOps platform can demand significant time and resources.
Factors like the variety of data sources, along with efficient data storage, security, and retention, play a critical role in the success of AIOps applications.
Potential Risks of Excessive Automation: Relying too heavily on automated processes can lead to a single failure point, diminishing the IT team's capacity to adapt and respond to new challenges.
Issues of Bias and Ethics in AI: Implementing AI technologies, including AIOps, brings the risk of bias and ethical dilemmas. These technologies may unintentionally perpetuate or even amplify existing biases present in the data sets.
Best AIOps vendors
To effectively showcase the benefits and minimize risks associated with deploying AIOps, organizations are advised to implement the technology in small, strategically planned phases.
It's important to select the right hosting model for the AIOps tool, whether on-premises or as a cloud-based service.
The IT team needs to comprehensively understand the system and then tailor it to fit the organization's specific requirements, ensuring a sufficient amount of data from the monitored systems.
This includes, but is not limited to, a variety of offerings in the market.
- BMC Software TrueSight.
- Cisco Crosswork Situation Manager.
- Datadog.
- Datapipe Trebuchet.
- Dynatrace.
- HCL Software Dryice.
- Moogsoft.
- New Relic.
- ServiceNow IT Service Management.
- Splunk IT Service Intelligence.
The outlook for AIOps is exceptionally bright. A study by The Insight Partners projects that the global AIOps platform market is set to grow from $2.83 billion in 2021 to a staggering $19.93 billion by 2028, marking a significant compound annual growth rate.
AIOps is poised to revolutionize enterprise IT operations by reducing noise, enhancing collaboration, providing comprehensive visibility, and elevating IT service management.
This technology is seen as a pivotal force in driving digital transformation, offering businesses a more agile, flexible, and secure IT infrastructure. Moreover, its integration into DevOps initiatives for automating infrastructure operations is expected to further its market acceptance and maturity.
Expanding on this, AIOps platforms have gained substantial traction in the enterprise realm, becoming indispensable for managing complex data environments and integrating across various ITOM functions.
The AIOps market itself is on a trajectory of rapid growth. According to Gartner, the market value is anticipated to hit around $2.1 billion by 2025, expanding at an impressive annual growth rate of about 19%.
Furthermore, Future Market Insights predicts that the AIOps platform market may reach a monumental $80.2 billion by 2032, with a CAGR of 25.4% from 2022 to 2032.
An interesting development in the field is the emergence of Chat GPT and generative AI, which are expected to play a crucial role in the evolution of AIOps. A TechTarget report highlights the potential applications of generative AI in tasks such as application code development and routine engineering tasks, including test generation.